Analizando o histórico do CVPR
Enquanto a IEEE/CVF Conference on Computer Vision and Pattern Recognition (CVPR) 2025 se aproxima, vamos dar uma olhada no histórico da conferência e de seus workshops de 2017 a 2024. O objetivo desta análise é proporcionar uma compreensão sobre a evolução dos tópicos e tendências na pesquisa de inteligência artificial ao longo dos anos. Tenha em mente que essas informações devem ser analisadas com cautela, pois alguns dados que poderiam ser relevantes para as análises são descartados durante o processo de limpeza. Parte da análise baseia-se em palavras-chave, e fazemos algumas suposições sobre como os autores as utilizam (por exemplo, é bastante improvável que um artigo sobre dados de imagem tenha a palavra-chave audio
em seu título ou resumo), mas essa não é uma solução perfeita. O objetivo desta postagem é fornecer uma visão sobre a história da conferência, e não uma análise definitiva.
Observe que alguns dos gráficos utilizam percentis do número total de artigos publicados a cada ano. Como há quantidades diferentes de artigos publicados a cada ano, os números não podem ser comparados diretamente de um ano para o outro. O objetivo desses gráficos é mostrar a distribuição dos artigos publicados durante o período e quaisquer mudanças no foco da comunidade acadêmica. Você também pode interagir com as visualizações. É possível dar zoom em partes específicas, habilitar ou desabilitar linhas clicando em seus nomes na legenda, e passar o mouse sobre os pontos para ver mais informações.
Estatísticas Gerais
Aqui você pode ver o número de artigos publicados. A cada ano, há mais e mais artigos publicados em comparação com o ano anterior, exceto em 2023. Foram publicados mais de três vezes mais artigos em 2024 do que em 2017.
{"data": [{"hovertemplate": "ano=%{x}<br>artigos=%{y}<extra></extra>", "legendgroup": "", "line": {"dash": "solid"}, "marker": {"symbol": "circle"}, "mode": "lines+markers", "name": "", "orientation": "v", "showlegend": false, "x": {"dtype": "i2", "bdata": "4QfiB+MH5AflB+YH5wfoBw=="}, "xaxis": "x", "y": {"dtype": "i2", "bdata": "KAQuBVQHwQd+CEgKNgmgDQ=="}, "yaxis": "y", "type": "scatter"}], "layout": {"xaxis": {"anchor": "y", "domain": [0.0, 1.0], "title": {"text": "ano"}}, "yaxis": {"anchor": "x", "domain": [0.0, 1.0], "title": {"text": "artigos"}}, "legend": {"tracegroupgap": 0}}}
Em relação às modalidades utilizadas nos artigos, podemos ver que a modalidade de imagem continua sendo a mais comum, mas o uso de texto e de múltiplas modalidades aumentou significativamente. A aplicação de fluxo óptico, grafos e informações de profundidade diminuiu nos últimos anos, enquanto o uso de partículas permaneceu relativamente estável.
{"data": [{"customdata": [["audio"], ["audio"], ["audio"], ["audio"], ["audio"], ["audio"], ["audio"], ["audio"]], "hovertemplate": "modalidade=%{customdata[0]}<br>ano=%{x}<br>ocorrências (%)=%{y:.3f}<extra></extra>", "legendgroup": "audio", "line": {"dash": "solid"}, "marker": {"symbol": "circle"}, "mode": "lines+markers", "name": "audio", "orientation": "v", "showlegend": true, "x": {"dtype": "i2", "bdata": "4QfiB+MH5AflB+YH5wfoBw=="}, "xaxis": "x", "y": {"dtype": "f8", "bdata": "2FBeQ3kN5T8dk/Uck/XsP30vhdy8DuE/g0lGn20u6D/N5tSIhffrP/WdjfrORvU/WASE4EIk+z+P78JB9PgAQA=="}, "yaxis": "y", "type": "scatter"}, {"customdata": [["depth"], ["depth"], ["depth"], ["depth"], ["depth"], ["depth"], ["depth"], ["depth"]], "hovertemplate": "modalidade=%{customdata[0]}<br>ano=%{x}<br>ocorrências (%)=%{y:.3f}<extra></extra>", "legendgroup": "depth", "line": {"dash": "solid"}, "marker": {"symbol": "circle"}, "mode": "lines+markers", "name": "depth", "orientation": "v", "showlegend": true, "x": {"dtype": "i2", "bdata": "4QfiB+MH5AflB+YH5wfoBw=="}, "xaxis": "x", "y": {"dtype": "f8", "bdata": "zB4we8DsE0Ak1egj1egTQER0/3wvhRhAQj1lr7AmFEDHDwNNB98TQMTkCmJyBRFAzGK7c/F4EUDBpv1kCWwPQA=="}, "yaxis": "y", "type": "scatter"}, {"customdata": [["graph"], ["graph"], ["graph"], ["graph"], ["graph"], ["graph"], ["graph"], ["graph"]], "hovertemplate": "modalidade=%{customdata[0]}<br>ano=%{x}<br>ocorrências (%)=%{y:.3f}<extra></extra>", "legendgroup": "graph", "line": {"dash": "solid"}, "marker": {"symbol": "circle"}, "mode": "lines+markers", "name": "graph", "orientation": "v", "showlegend": true, "x": {"dtype": "i2", "bdata": "4QfiB+MH5AflB+YH5wfoBw=="}, "xaxis": "x", "y": {"dtype": "f8", "bdata": "HX1z9M3RC0A+eSo+eSoOQHumE2xSRRFAg0lGn20uGEDucuibFmAQQL+zUd/ZqAtALkSykbMfCkCWwKb9ZAkDQA=="}, "yaxis": "y", "type": "scatter"}, {"customdata": [["image"], ["image"], ["image"], ["image"], ["image"], ["image"], ["image"], ["image"]], "hovertemplate": "modalidade=%{customdata[0]}<br>ano=%{x}<br>ocorrências (%)=%{y:.3f}<extra></extra>", "legendgroup": "image", "line": {"dash": "solid"}, "marker": {"symbol": "circle"}, "mode": "lines+markers", "name": "image", "orientation": "v", "showlegend": true, "x": {"dtype": "i2", "bdata": "4QfiB+MH5AflB+YH5wfoBw=="}, "xaxis": "x", "y": {"dtype": "f8", "bdata": "eQ3lNZQXQkBUyixUyixCQAEGofVGhkBAMA4SdHz7PkB3eggk2Ro8QPeySqCiNz1AeiSI92T9O0B8Fw6ixydAQA=="}, "yaxis": "y", "type": "scatter"}, {"customdata": [["mesh"], ["mesh"], ["mesh"], ["mesh"], ["mesh"], ["mesh"], ["mesh"], ["mesh"]], "hovertemplate": "modalidade=%{customdata[0]}<br>ano=%{x}<br>ocorrências (%)=%{y:.3f}<extra></extra>", "legendgroup": "mesh", "line": {"dash": "solid"}, "marker": {"symbol": "circle"}, "mode": "lines+markers", "name": "mesh", "orientation": "v", "showlegend": true, "x": {"dtype": "i2", "bdata": "4QfiB+MH5AflB+YH5wfoBw=="}, "xaxis": "x", "y": {"dtype": "f8", "bdata": "9oDZA2YP6D/bxovaxovqP0kPVM5u4fc/kOUMnMb8+D9dS0BRmUsBQCZXEJMriPk/XoOZB+cIBUCUJbBpP1kCQA=="}, "yaxis": "y", "type": "scatter"}, {"customdata": [["multi modal"], ["multi modal"], ["multi modal"], ["multi modal"], ["multi modal"], ["multi modal"], ["multi modal"], ["multi modal"]], "hovertemplate": "modalidade=%{customdata[0]}<br>ano=%{x}<br>ocorrências (%)=%{y:.3f}<extra></extra>", "legendgroup": "multi modal", "line": {"dash": "solid"}, "marker": {"symbol": "circle"}, "mode": "lines+markers", "name": "multi modal", "orientation": "v", "showlegend": true, "x": {"dtype": "i2", "bdata": "4QfiB+MH5AflB+YH5wfoBw=="}, "xaxis": "x", "y": {"dtype": "f8", "bdata": "FbFUxFIRC0A1SIM0SIMEQFJF/XDtmQZAw1Unjyo2DEDlDfuqVnANQCdeT8ocAxhA1CNBvCfrF0DtJ0tg034jQA=="}, "yaxis": "y", "type": "scatter"}, {"customdata": [["optical flow"], ["optical flow"], ["optical flow"], ["optical flow"], ["optical flow"], ["optical flow"], ["optical flow"], ["optical flow"]], "hovertemplate": "modalidade=%{customdata[0]}<br>ano=%{x}<br>ocorrências (%)=%{y:.3f}<extra></extra>", "legendgroup": "optical flow", "line": {"dash": "solid"}, "marker": {"symbol": "circle"}, "mode": "lines+markers", "name": "optical flow", "orientation": "v", "showlegend": true, "x": {"dtype": "i2", "bdata": "4QfiB+MH5AflB+YH5wfoBw=="}, "xaxis": "x", "y": {"dtype": "f8", "bdata": "yLgg44KMA0A+eSo+eSr+Pw7LXP52jv8/98VBgo5v/z+EcWIiEo33P1osjwhNtfc/JoMsSX2t8z8Vc6szUjHvPw=="}, "yaxis": "y", "type": "scatter"}, {"customdata": [["particle"], ["particle"], ["particle"], ["particle"], ["particle"], ["particle"], ["particle"], ["particle"]], "hovertemplate": "modalidade=%{customdata[0]}<br>ano=%{x}<br>ocorrências (%)=%{y:.3f}<extra></extra>", "legendgroup": "particle", "line": {"dash": "solid"}, "marker": {"symbol": "circle"}, "mode": "lines+markers", "name": "particle", "orientation": "v", "showlegend": true, "x": {"dtype": "i2", "bdata": "4QfiB+MH5AflB+YH5wfoBw=="}, "xaxis": "x", "y": {"dtype": "f8", "bdata": "9oDZA2YPyD8TYk4TYk6zPy5/O8fHSrs/AjGEv/Me0D8j1cmZzanRPylzDHDwc9M/mwIc7fRIwD84iB7fhYPQPw=="}, "yaxis": "y", "type": "scatter"}, {"customdata": [["path"], ["path"], ["path"], ["path"], ["path"], ["path"], ["path"], ["path"]], "hovertemplate": "modalidade=%{customdata[0]}<br>ano=%{x}<br>ocorrências (%)=%{y:.3f}<extra></extra>", "legendgroup": "path", "line": {"dash": "solid"}, "marker": {"symbol": "circle"}, "mode": "lines+markers", "name": "path", "orientation": "v", "showlegend": true, "x": {"dtype": "i2", "bdata": "4QfiB+MH5AflB+YH5wfoBw=="}, "xaxis": "x", "y": {"dtype": "f8", "bdata": "NOHPhD8T7j+Y+iGY+iH4P4RTS55mNABAg0lGn20u+D+cmIhE4wX5P1osjwhNtfc/QgNjKDJb9D9GKuZWZ6T0Pw=="}, "yaxis": "y", "type": "scatter"}, {"customdata": [["point cloud"], ["point cloud"], ["point cloud"], ["point cloud"], ["point cloud"], ["point cloud"], ["point cloud"], ["point cloud"]], "hovertemplate": "modalidade=%{customdata[0]}<br>ano=%{x}<br>ocorrências (%)=%{y:.3f}<extra></extra>", "legendgroup": "point cloud", "line": {"dash": "solid"}, "marker": {"symbol": "circle"}, "mode": "lines+markers", "name": "point cloud", "orientation": "v", "showlegend": true, "x": {"dtype": "i2", "bdata": "4QfiB+MH5AflB+YH5wfoBw=="}, "xaxis": "x", "y": {"dtype": "f8", "bdata": "FbFUxFIR6z8D77MC77MCQFlpwzKXvwVAw1Unjyo2DED9NCHNJ+kOQCVQ0Vs6DQtAA2MoMlvUEkCpmFudkYoIQA=="}, "yaxis": "y", "type": "scatter"}, {"customdata": [["text"], ["text"], ["text"], ["text"], ["text"], ["text"], ["text"], ["text"]], "hovertemplate": "modalidade=%{customdata[0]}<br>ano=%{x}<br>ocorrências (%)=%{y:.3f}<extra></extra>", "legendgroup": "text", "line": {"dash": "solid"}, "marker": {"symbol": "circle"}, "mode": "lines+markers", "name": "text", "orientation": "v", "showlegend": true, "x": {"dtype": "i2", "bdata": "4QfiB+MH5AflB+YH5wfoBw=="}, "xaxis": "x", "y": {"dtype": "f8", "bdata": "9oDZA2YPGEB3FO12FO0WQD/ZqivwKBlAU4Bd658oFUBUIxbeb5sUQChljgEOfhZAQgNjKDJbFEAJbNpPlsAbQA=="}, "yaxis": "y", "type": "scatter"}, {"customdata": [["video"], ["video"], ["video"], ["video"], ["video"], ["video"], ["video"], ["video"]], "hovertemplate": "modalidade=%{customdata[0]}<br>ano=%{x}<br>ocorrências (%)=%{y:.3f}<extra></extra>", "legendgroup": "video", "line": {"dash": "solid"}, "marker": {"symbol": "circle"}, "mode": "lines+markers", "name": "video", "orientation": "v", "showlegend": true, "x": {"dtype": "i2", "bdata": "4QfiB+MH5AflB+YH5wfoBw=="}, "xaxis": "x", "y": {"dtype": "f8", "bdata": "NpTXUF5DLkCE5g2E5g0sQMnTjAYkxidAjT7bXDDJKEDsq1rBdfQqQL+zUd/ZqCtANeR/ySBLKkDYtJ8sgc0pQA=="}, "yaxis": "y", "type": "scatter"}], "layout": {"legend": {"title": {"text": "modalidade"}, "tracegroupgap": 0}, "xaxis": {"anchor": "y", "domain": [0.0, 1.0], "title": {"text": "ano"}}, "yaxis": {"anchor": "x", "domain": [0.0, 1.0], "title": {"text": "ocorrências (%)"}}}}
É bastante comum que os artigos introduzam novos conceitos, seja um novo método, um novo conjunto de dados ou uma nova arquitetura. O gráfico a seguir mostra os conceitos mais comuns apresentados nos artigos. Não é surpreendente que algoritmos sejam o conceito mais frequente. Algoritmos também envolvem novos métodos ou abordagens. Novas tarefas foram introduzidas ao longo dos anos, o que está altamente correlacionado com a criação de novos conjuntos de dados. A introdução de novas arquiteturas também aumentou no último ano, incluindo novos modelos, módulos e redes. A criação de diferentes funções de perda e métricas tem se mantido bastante estável ao longo dos anos, com pouquíssimos artigos introduzindo novidades.
{"data": [{"customdata": [["algorithms"], ["algorithms"], ["algorithms"], ["algorithms"], ["algorithms"], ["algorithms"], ["algorithms"], ["algorithms"]], "hovertemplate": "conceito=%{customdata[0]}<br>ano=%{x}<br>ocorrências (%)=%{y:.3f}<extra></extra>", "legendgroup": "algorithms", "line": {"dash": "solid"}, "marker": {"symbol": "circle"}, "mode": "lines+markers", "name": "algorithms", "orientation": "v", "showlegend": true, "x": {"dtype": "i2", "bdata": "4QfiB+MH5AflB+YH5wfoBw=="}, "xaxis": "x", "y": {"dtype": "f8", "bdata": "qYilIpaKAEA+eSo+eSr+P1x7phNsUgVA3Y20iNzS/T9UIxbeb5v0P1osjwhNtfc/GoUB+zTk/z+P78JB9PgQQA=="}, "yaxis": "y", "type": "scatter"}, {"customdata": [["architectures"], ["architectures"], ["architectures"], ["architectures"], ["architectures"], ["architectures"], ["architectures"], ["architectures"]], "hovertemplate": "conceito=%{customdata[0]}<br>ano=%{x}<br>ocorrências (%)=%{y:.3f}<extra></extra>", "legendgroup": "architectures", "line": {"dash": "solid"}, "marker": {"symbol": "circle"}, "mode": "lines+markers", "name": "architectures", "orientation": "v", "showlegend": true, "x": {"dtype": "i2", "bdata": "4QfiB+MH5AflB+YH5wfoBw=="}, "xaxis": "x", "y": {"dtype": "f8", "bdata": "2FBeQ3kN5T9fX19fX1/vP4RTS55mNPA/0PHti4ME7T+XwbYIZe3wPylzDHDwc/M//gTLG4A27z8H0eO7cBD7Pw=="}, "yaxis": "y", "type": "scatter"}, {"customdata": [["datasets"], ["datasets"], ["datasets"], ["datasets"], ["datasets"], ["datasets"], ["datasets"], ["datasets"]], "hovertemplate": "conceito=%{customdata[0]}<br>ano=%{x}<br>ocorrências (%)=%{y:.3f}<extra></extra>", "legendgroup": "datasets", "line": {"dash": "solid"}, "marker": {"symbol": "circle"}, "mode": "lines+markers", "name": "datasets", "orientation": "v", "showlegend": true, "x": {"dtype": "i2", "bdata": "4QfiB+MH5AflB+YH5wfoBw=="}, "xaxis": "x", "y": {"dtype": "f8", "bdata": "5+ibo2+O9j/8rMD7rMD7PyE3r0N0//w/g0lGn20u+D87/O+7niLzP4rXkzLHAP8/44SUPMuI/j9s2k+WwKb/Pw=="}, "yaxis": "y", "type": "scatter"}, {"customdata": [["losses"], ["losses"], ["losses"], ["losses"], ["losses"], ["losses"], ["losses"], ["losses"]], "hovertemplate": "conceito=%{customdata[0]}<br>ano=%{x}<br>ocorrências (%)=%{y:.3f}<extra></extra>", "legendgroup": "losses", "line": {"dash": "solid"}, "marker": {"symbol": "circle"}, "mode": "lines+markers", "name": "losses", "orientation": "v", "showlegend": true, "x": {"dtype": "i2", "bdata": "4QfiB+MH5AflB+YH5wfoBw=="}, "xaxis": "x", "y": {"dtype": "f8", "bdata": "9oDZA2YPuD8dk/Uck/XcP30vhdy8DtE/aRG5pbuR1j+1v65mtH7aP76sEqjoLc0/WASE4EIkyz9LYNN+sgTGPw=="}, "yaxis": "y", "type": "scatter"}, {"customdata": [["metrics"], ["metrics"], ["metrics"], ["metrics"], ["metrics"], ["metrics"], ["metrics"], ["metrics"]], "hovertemplate": "conceito=%{customdata[0]}<br>ano=%{x}<br>ocorrências (%)=%{y:.3f}<extra></extra>", "legendgroup": "metrics", "line": {"dash": "solid"}, "marker": {"symbol": "circle"}, "mode": "lines+markers", "name": "metrics", "orientation": "v", "showlegend": true, "x": {"dtype": "i2", "bdata": "4QfiB+MH5AflB+YH5wfoBw=="}, "xaxis": "x", "y": {"dtype": "f8", "bdata": "AAAAAAAAAAATYk4TYk6zPy5/O8fHSqs/nYHTmB/LuT/lDfuqVnDNPwAAAAAAAAAAeQPQ5pu2pT+61RmpmFu9Pw=="}, "yaxis": "y", "type": "scatter"}, {"customdata": [["tasks"], ["tasks"], ["tasks"], ["tasks"], ["tasks"], ["tasks"], ["tasks"], ["tasks"]], "hovertemplate": "conceito=%{customdata[0]}<br>ano=%{x}<br>ocorrências (%)=%{y:.3f}<extra></extra>", "legendgroup": "tasks", "line": {"dash": "solid"}, "marker": {"symbol": "circle"}, "mode": "lines+markers", "name": "tasks", "orientation": "v", "showlegend": true, "x": {"dtype": "i2", "bdata": "4QfiB+MH5AflB+YH5wfoBw=="}, "xaxis": "x", "y": {"dtype": "f8", "bdata": "9oDZA2YP2D8TYk4TYk7TPxTvIsAgtO4/0PHti4ME7T9sSjwAQRTmP1w6DXcvq/Q/XoOZB+cI9T9GKuZWZ6T0Pw=="}, "yaxis": "y", "type": "scatter"}], "layout": {"xaxis": {"anchor": "y", "domain": [0.0, 1.0], "title": {"text": "ano"}}, "yaxis": {"anchor": "x", "domain": [0.0, 1.0], "title": {"text": "ocorrências (%)"}}, "legend": {"title": {"text": "conceito"}, "tracegroupgap": 0}}}
Em relação às tarefas comuns nos artigos, podemos observar um grande aumento nas tarefas de geração, especialmente após 2022. Isso pode estar relacionado aos avanços em grandes modelos de linguagem, como InstructGPT e ChatGPT no final de 2022, e ao lançamento das primeiras coleções de modelos fundamentais de linguagem, como o LLaMA no início de 2023. Classificação, detecção, estimação e reconhecimento têm apresentado uma queda de interesse ao longo dos anos, enquanto previsão só apresentou diminuição recentemente. Tarefas como segmentação permaneceram relativamente estáveis. O uso de tarefas de raciocínio também aumentou significativamente no último ano, mas ainda corresponde a uma pequena porcentagem do total de artigos publicados (cerca de 3%).
{"data": [{"customdata": [["captioning"], ["captioning"], ["captioning"], ["captioning"], ["captioning"], ["captioning"], ["captioning"], ["captioning"]], "hovertemplate": "tarefa=%{customdata[0]}<br>ano=%{x}<br>ocorrências (%)=%{y:.3f}<extra></extra>", "legendgroup": "captioning", "line": {"dash": "solid"}, "marker": {"symbol": "circle"}, "mode": "lines+markers", "name": "captioning", "orientation": "v", "showlegend": true, "x": {"dtype": "i2", "bdata": "4QfiB+MH5AflB+YH5wfoBw=="}, "xaxis": "x", "y": {"dtype": "f8", "bdata": "JUmSJEmS/D/yexnyexnyP3YLvxoT6fE/T9krrAn15D9UIxbeb5vkP76sEqjoLe0/mwIc7fRI8D/5LhxEj+/2Pw=="}, "yaxis": "y", "type": "scatter"}, {"customdata": [["classification"], ["classification"], ["classification"], ["classification"], ["classification"], ["classification"], ["classification"], ["classification"]], "hovertemplate": "tarefa=%{customdata[0]}<br>ano=%{x}<br>ocorrências (%)=%{y:.3f}<extra></extra>", "legendgroup": "classification", "line": {"dash": "solid"}, "marker": {"symbol": "circle"}, "mode": "lines+markers", "name": "classification", "orientation": "v", "showlegend": true, "x": {"dtype": "i2", "bdata": "4QfiB+MH5AflB+YH5wfoBw=="}, "xaxis": "x", "y": {"dtype": "f8", "bdata": "zB4we8DsI0CmpaWlpaUhQPI0owGJcSJAlEcVG646IUCng+85dnYfQL+zUd/ZqBtAzYNzhLq/F0DMrc5IxVwWQA=="}, "yaxis": "y", "type": "scatter"}, {"customdata": [["clustering"], ["clustering"], ["clustering"], ["clustering"], ["clustering"], ["clustering"], ["clustering"], ["clustering"]], "hovertemplate": "tarefa=%{customdata[0]}<br>ano=%{x}<br>ocorrências (%)=%{y:.3f}<extra></extra>", "legendgroup": "clustering", "line": {"dash": "solid"}, "marker": {"symbol": "circle"}, "mode": "lines+markers", "name": "clustering", "orientation": "v", "showlegend": true, "x": {"dtype": "i2", "bdata": "4QfiB+MH5AflB+YH5wfoBw=="}, "xaxis": "x", "y": {"dtype": "f8", "bdata": "qYilIpaKAED8rMD7rMD7P4RTS55mNABAIrd0N9IiAkDRNy3AMI8AQF1Ii+URoQFA/gTLG4A2/z9VzK3OSMX4Pw=="}, "yaxis": "y", "type": "scatter"}, {"customdata": [["counting"], ["counting"], ["counting"], ["counting"], ["counting"], ["counting"], ["counting"], ["counting"]], "hovertemplate": "tarefa=%{customdata[0]}<br>ano=%{x}<br>ocorrências (%)=%{y:.3f}<extra></extra>", "legendgroup": "counting", "line": {"dash": "solid"}, "marker": {"symbol": "circle"}, "mode": "lines+markers", "name": "counting", "orientation": "v", "showlegend": true, "x": {"dtype": "i2", "bdata": "4QfiB+MH5AflB+YH5wfoBw=="}, "xaxis": "x", "y": {"dtype": "f8", "bdata": "2FBeQ3kN5T9WLrhVLrjlP1x7phNsUvU/0PHti4ME7T9sSjwAQRTmP44BDn5u4uU/6QOqY29t2D8Vc6szUjHfPw=="}, "yaxis": "y", "type": "scatter"}, {"customdata": [["detection"], ["detection"], ["detection"], ["detection"], ["detection"], ["detection"], ["detection"], ["detection"]], "hovertemplate": "tarefa=%{customdata[0]}<br>ano=%{x}<br>ocorrências (%)=%{y:.3f}<extra></extra>", "legendgroup": "detection", "line": {"dash": "solid"}, "marker": {"symbol": "circle"}, "mode": "lines+markers", "name": "detection", "orientation": "v", "showlegend": true, "x": {"dtype": "i2", "bdata": "4QfiB+MH5AflB+YH5wfoBw=="}, "xaxis": "x", "y": {"dtype": "f8", "bdata": "DDIuyLggKkCIh4eHh4cnQMOvxkR6oChAFWDX+idKKUCJhffbJuUlQNuvLqTF8iZAA2MoMlvUIkB/sgQ27WciQA=="}, "yaxis": "y", "type": "scatter"}, {"customdata": [["estimation"], ["estimation"], ["estimation"], ["estimation"], ["estimation"], ["estimation"], ["estimation"], ["estimation"]], "hovertemplate": "tarefa=%{customdata[0]}<br>ano=%{x}<br>ocorrências (%)=%{y:.3f}<extra></extra>", "legendgroup": "estimation", "line": {"dash": "solid"}, "marker": {"symbol": "circle"}, "mode": "lines+markers", "name": "estimation", "orientation": "v", "showlegend": true, "x": {"dtype": "i2", "bdata": "4QfiB+MH5AflB+YH5wfoBw=="}, "xaxis": "x", "y": {"dtype": "f8", "bdata": "vzn65uibIkA/Uo0+Uo0iQBycWvI0ox1AEnR8++IgIUAfhHFiIhIdQCZXEJMriBlAPIRNAY52GkD7yRLYtJ8XQA=="}, "yaxis": "y", "type": "scatter"}, {"customdata": [["forecasting"], ["forecasting"], ["forecasting"], ["forecasting"], ["forecasting"], ["forecasting"], ["forecasting"], ["forecasting"]], "hovertemplate": "tarefa=%{customdata[0]}<br>ano=%{x}<br>ocorrências (%)=%{y:.3f}<extra></extra>", "legendgroup": "forecasting", "line": {"dash": "solid"}, "marker": {"symbol": "circle"}, "mode": "lines+markers", "name": "forecasting", "orientation": "v", "showlegend": true, "x": {"dtype": "i2", "bdata": "4QfiB+MH5AflB+YH5wfoBw=="}, "xaxis": "x", "y": {"dtype": "f8", "bdata": "9oDZA2YP2D8TYk4TYk7TP0kPVM5u4dc/g0lGn20u6D87/O+7niLjP/BzE68nZe4/0wKJq16k4T8Cm/aTJbDpPw=="}, "yaxis": "y", "type": "scatter"}, {"customdata": [["generation"], ["generation"], ["generation"], ["generation"], ["generation"], ["generation"], ["generation"], ["generation"]], "hovertemplate": "tarefa=%{customdata[0]}<br>ano=%{x}<br>ocorrências (%)=%{y:.3f}<extra></extra>", "legendgroup": "generation", "line": {"dash": "solid"}, "marker": {"symbol": "circle"}, "mode": "lines+markers", "name": "generation", "orientation": "v", "showlegend": true, "x": {"dtype": "i2", "bdata": "4QfiB+MH5AflB+YH5wfoBw=="}, "xaxis": "x", "y": {"dtype": "f8", "bdata": "+ubom6NvGECEv3CEv3AgQHumE2xSRSFAzgWTjD7bJEBNhbbHUBcnQPSPD4zsUChAtRpffs7rMECuzkjF3Mo2QA=="}, "yaxis": "y", "type": "scatter"}, {"customdata": [["identification"], ["identification"], ["identification"], ["identification"], ["identification"], ["identification"], ["identification"], ["identification"]], "hovertemplate": "tarefa=%{customdata[0]}<br>ano=%{x}<br>ocorrências (%)=%{y:.3f}<extra></extra>", "legendgroup": "identification", "line": {"dash": "solid"}, "marker": {"symbol": "circle"}, "mode": "lines+markers", "name": "identification", "orientation": "v", "showlegend": true, "x": {"dtype": "i2", "bdata": "4QfiB+MH5AflB+YH5wfoBw=="}, "xaxis": "x", "y": {"dtype": "f8", "bdata": "wOwBswfMAkAMIFsMIFsMQDWjAYlxcApAQj1lr7AmBEA7/O+7niIDQPKBkR0KW/s/WASE4EIk6z9LYNN+sgT2Pw=="}, "yaxis": "y", "type": "scatter"}, {"customdata": [["navigation"], ["navigation"], ["navigation"], ["navigation"], ["navigation"], ["navigation"], ["navigation"], ["navigation"]], "hovertemplate": "tarefa=%{customdata[0]}<br>ano=%{x}<br>ocorrências (%)=%{y:.3f}<extra></extra>", "legendgroup": "navigation", "line": {"dash": "solid"}, "marker": {"symbol": "circle"}, "mode": "lines+markers", "name": "navigation", "orientation": "v", "showlegend": true, "x": {"dtype": "i2", "bdata": "4QfiB+MH5AflB+YH5wfoBw=="}, "xaxis": "x", "y": {"dtype": "f8", "bdata": "9oDZA2YP6D93FO12FO32P0kPVM5u4fc/trlgktFn6z/HDwNNB9/zPylzDHDwc/M/7oK/ihNS8j+ix3fhIHr2Pw=="}, "yaxis": "y", "type": "scatter"}, {"customdata": [["prediction"], ["prediction"], ["prediction"], ["prediction"], ["prediction"], ["prediction"], ["prediction"], ["prediction"]], "hovertemplate": "tarefa=%{customdata[0]}<br>ano=%{x}<br>ocorrências (%)=%{y:.3f}<extra></extra>", "legendgroup": "prediction", "line": {"dash": "solid"}, "marker": {"symbol": "circle"}, "mode": "lines+markers", "name": "prediction", "orientation": "v", "showlegend": true, "x": {"dtype": "i2", "bdata": "4QfiB+MH5AflB+YH5wfoBw=="}, "xaxis": "x", "y": {"dtype": "f8", "bdata": "tbrT6k6rIUAdbFgdbFghQOBRwiz2ySRA6+RRxYarJkA7/O+7niIjQEFMriAmVyZA76N3m9yYKEDDQfT4LpwjQA=="}, "yaxis": "y", "type": "scatter"}, {"customdata": [["reasoning"], ["reasoning"], ["reasoning"], ["reasoning"], ["reasoning"], ["reasoning"], ["reasoning"], ["reasoning"]], "hovertemplate": "tarefa=%{customdata[0]}<br>ano=%{x}<br>ocorrências (%)=%{y:.3f}<extra></extra>", "legendgroup": "reasoning", "line": {"dash": "solid"}, "marker": {"symbol": "circle"}, "mode": "lines+markers", "name": "reasoning", "orientation": "v", "showlegend": true, "x": {"dtype": "i2", "bdata": "4QfiB+MH5AflB+YH5wfoBw=="}, "xaxis": "x", "y": {"dtype": "f8", "bdata": "2FBeQ3kNBUAk1egj1egDQFJF/XDtmQZAfPviIEHHB0DWDv/7rqcIQMPWjPOPDwRAJoMsSX2tA0AJbNpPlsALQA=="}, "yaxis": "y", "type": "scatter"}, {"customdata": [["recognition"], ["recognition"], ["recognition"], ["recognition"], ["recognition"], ["recognition"], ["recognition"], ["recognition"]], "hovertemplate": "tarefa=%{customdata[0]}<br>ano=%{x}<br>ocorrências (%)=%{y:.3f}<extra></extra>", "legendgroup": "recognition", "line": {"dash": "solid"}, "marker": {"symbol": "circle"}, "mode": "lines+markers", "name": "recognition", "orientation": "v", "showlegend": true, "x": {"dtype": "i2", "bdata": "4QfiB+MH5AflB+YH5wfoBw=="}, "xaxis": "x", "y": {"dtype": "f8", "bdata": "2FBeQ3kNJUCmpaWlpaUhQCbSA5WzWxxAumCS0WebG0D4XU+RqdAWQPSPD4zsUBhAQgNjKDJbFEBlCWzaTxYRQA=="}, "yaxis": "y", "type": "scatter"}, {"customdata": [["regression"], ["regression"], ["regression"], ["regression"], ["regression"], ["regression"], ["regression"], ["regression"]], "hovertemplate": "tarefa=%{customdata[0]}<br>ano=%{x}<br>ocorrências (%)=%{y:.3f}<extra></extra>", "legendgroup": "regression", "line": {"dash": "solid"}, "marker": {"symbol": "circle"}, "mode": "lines+markers", "name": "regression", "orientation": "v", "showlegend": true, "x": {"dtype": "i2", "bdata": "4QfiB+MH5AflB+YH5wfoBw=="}, "xaxis": "x", "y": {"dtype": "f8", "bdata": "LBWxVMRSDUAk1egj1egDQHDn+FhpwwJAKQXYtf6JAkAj1cmZzakBQPakzDHAwQNAQgNjKDJb9D+n/WQJbNr3Pw=="}, "yaxis": "y", "type": "scatter"}, {"customdata": [["retrieval"], ["retrieval"], ["retrieval"], ["retrieval"], ["retrieval"], ["retrieval"], ["retrieval"], ["retrieval"]], "hovertemplate": "tarefa=%{customdata[0]}<br>ano=%{x}<br>ocorrências (%)=%{y:.3f}<extra></extra>", "legendgroup": "retrieval", "line": {"dash": "solid"}, "marker": {"symbol": "circle"}, "mode": "lines+markers", "name": "retrieval", "orientation": "v", "showlegend": true, "x": {"dtype": "i2", "bdata": "4QfiB+MH5AflB+YH5wfoBw=="}, "xaxis": "x", "y": {"dtype": "f8", "bdata": "DeU1lNdQCkBWLrhVLrgFQFJF/XDtmQZAL1M7NCvxAkAQhXWzekkIQFszzj8+MAZASsTocGjNCkDyXTiIHt8EQA=="}, "yaxis": "y", "type": "scatter"}, {"customdata": [["segmentation"], ["segmentation"], ["segmentation"], ["segmentation"], ["segmentation"], ["segmentation"], ["segmentation"], ["segmentation"]], "hovertemplate": "tarefa=%{customdata[0]}<br>ano=%{x}<br>ocorrências (%)=%{y:.3f}<extra></extra>", "legendgroup": "segmentation", "line": {"dash": "solid"}, "marker": {"symbol": "circle"}, "mode": "lines+markers", "name": "segmentation", "orientation": "v", "showlegend": true, "x": {"dtype": "i2", "bdata": "4QfiB+MH5AflB+YH5wfoBw=="}, "xaxis": "x", "y": {"dtype": "f8", "bdata": "MHvA7AGzHUDRleTQleQgQAOPEmaxTyBAgF3rnygFIECrl4Tzis4dQF5PyhwDHCBAmwIc7fRIIEDPSMXc6swgQA=="}, "yaxis": "y", "type": "scatter"}, {"customdata": [["tracking"], ["tracking"], ["tracking"], ["tracking"], ["tracking"], ["tracking"], ["tracking"], ["tracking"]], "hovertemplate": "tarefa=%{customdata[0]}<br>ano=%{x}<br>ocorrências (%)=%{y:.3f}<extra></extra>", "legendgroup": "tracking", "line": {"dash": "solid"}, "marker": {"symbol": "circle"}, "mode": "lines+markers", "name": "tracking", "orientation": "v", "showlegend": true, "x": {"dtype": "i2", "bdata": "4QfiB+MH5AflB+YH5wfoBw=="}, "xaxis": "x", "y": {"dtype": "f8", "bdata": "0IQ/E/5MFEBJXJdIXJcQQChbdQUeJQxAtrlgktFnC0DucuibFmAQQCVQ0Vs6DQtAQgNjKDJbBEAFNu0nS2AKQA=="}, "yaxis": "y", "type": "scatter"}, {"customdata": [["translation"], ["translation"], ["translation"], ["translation"], ["translation"], ["translation"], ["translation"], ["translation"]], "hovertemplate": "tarefa=%{customdata[0]}<br>ano=%{x}<br>ocorrências (%)=%{y:.3f}<extra></extra>", "legendgroup": "translation", "line": {"dash": "solid"}, "marker": {"symbol": "circle"}, "mode": "lines+markers", "name": "translation", "orientation": "v", "showlegend": true, "x": {"dtype": "i2", "bdata": "4QfiB+MH5AflB+YH5wfoBw=="}, "xaxis": "x", "y": {"dtype": "f8", "bdata": "FbFUxFIR6z8TYk4TYk4DQFJF/XDtmQZAPO8BMYS/A0BUIxbeb5sEQJEWyyNCUwFAlYMGxlBk9j+ZW52RirnzPw=="}, "yaxis": "y", "type": "scatter"}, {"customdata": [["verification"], ["verification"], ["verification"], ["verification"], ["verification"], ["verification"], ["verification"], ["verification"]], "hovertemplate": "tarefa=%{customdata[0]}<br>ano=%{x}<br>ocorrências (%)=%{y:.3f}<extra></extra>", "legendgroup": "verification", "line": {"dash": "solid"}, "marker": {"symbol": "circle"}, "mode": "lines+markers", "name": "verification", "orientation": "v", "showlegend": true, "x": {"dtype": "i2", "bdata": "4QfiB+MH5AflB+YH5wfoBw=="}, "xaxis": "x", "y": {"dtype": "f8", "bdata": "FbFUxFIR6z8dk/Uck/XsPxTvIsAgtO4/g0lGn20u6D+EcWIiEo3XP/SPD4zsUNg/eQPQ5pu21T84iB7fhYPQPw=="}, "yaxis": "y", "type": "scatter"}], "layout": {"xaxis": {"anchor": "y", "domain": [0.0, 1.0], "title": {"text": "ano"}}, "yaxis": {"anchor": "x", "domain": [0.0, 1.0], "title": {"text": "ocorrências (%)"}}, "legend": {"title": {"text": "tarefa"}, "tracegroupgap": 0}}}
Vamos nos aprofundar um pouco mais nas tarefas.
Algoritmos focados em segurança e privacidade existem há algum tempo, mas o número de artigos publicados sobre eles aumentou significativamente no último ano. A detecção de spoofing é crucial para aplicações como o reconhecimento de identidade, onde atacantes podem tentar utilizar fotos ou vídeos para se fazer passar por outra pessoa, e parece ter ganhado urgência desde que as tecnologias deepfake se tornaram mais prevalentes.
{"data": [{"customdata": [["adversarial attack"], ["adversarial attack"], ["adversarial attack"], ["adversarial attack"], ["adversarial attack"], ["adversarial attack"], ["adversarial attack"], ["adversarial attack"]], "hovertemplate": "tarefa=%{customdata[0]}<br>ano=%{x}<br>ocorrências (%)=%{y:.3f}<extra></extra>", "legendgroup": "adversarial attack", "line": {"dash": "solid"}, "marker": {"symbol": "circle"}, "mode": "lines+markers", "name": "adversarial attack", "orientation": "v", "showlegend": true, "x": {"dtype": "i2", "bdata": "4QfiB+MH5AflB+YH5wfoBw=="}, "xaxis": "x", "y": {"dtype": "f8", "bdata": "9oDZA2YPuD8dk/Uck/XcP1ZX4FHCLPY/D81KvEztAEBUIxbeb5v0P8HIDoWtGfc/BITgQiQb+T/5LhxEj+/2Pw=="}, "yaxis": "y", "type": "scatter"}, {"customdata": [["anomaly detection"], ["anomaly detection"], ["anomaly detection"], ["anomaly detection"], ["anomaly detection"], ["anomaly detection"], ["anomaly detection"], ["anomaly detection"]], "hovertemplate": "tarefa=%{customdata[0]}<br>ano=%{x}<br>ocorrências (%)=%{y:.3f}<extra></extra>", "legendgroup": "anomaly detection", "line": {"dash": "solid"}, "marker": {"symbol": "circle"}, "mode": "lines+markers", "name": "anomaly detection", "orientation": "v", "showlegend": true, "x": {"dtype": "i2", "bdata": "4QfiB+MH5AflB+YH5wfoBw=="}, "xaxis": "x", "y": {"dtype": "f8", "bdata": "9oDZA2YPyD8TYk4TYk7jP3Dn+Fhpw+I/aRG5pbuR5j8j1cmZzanhPylzDHDwc+M/mwIc7fRI4D8Vc6szUjHvPw=="}, "yaxis": "y", "type": "scatter"}, {"customdata": [["disambiguation"], ["disambiguation"], ["disambiguation"], ["disambiguation"], ["disambiguation"], ["disambiguation"], ["disambiguation"], ["disambiguation"]], "hovertemplate": "tarefa=%{customdata[0]}<br>ano=%{x}<br>ocorrências (%)=%{y:.3f}<extra></extra>", "legendgroup": "disambiguation", "line": {"dash": "solid"}, "marker": {"symbol": "circle"}, "mode": "lines+markers", "name": "disambiguation", "orientation": "v", "showlegend": true, "x": {"dtype": "i2", "bdata": "4QfiB+MH5AflB+YH5wfoBw=="}, "xaxis": "x", "y": {"dtype": "f8", "bdata": "9oDZA2YPuD8AAAAAAAAAAC5/O8fHSrs/AAAAAAAAAACEcWIiEo2nPylzDHDwc6M/mwIc7fRIwD+UJbBpP1nCPw=="}, "yaxis": "y", "type": "scatter"}, {"customdata": [["face verification"], ["face verification"], ["face verification"], ["face verification"], ["face verification"], ["face verification"], ["face verification"], ["face verification"]], "hovertemplate": "tarefa=%{customdata[0]}<br>ano=%{x}<br>ocorrências (%)=%{y:.3f}<extra></extra>", "legendgroup": "face verification", "line": {"dash": "solid"}, "marker": {"symbol": "circle"}, "mode": "lines+markers", "name": "face verification", "orientation": "v", "showlegend": true, "x": {"dtype": "i2", "bdata": "4QfiB+MH5AflB+YH5wfoBw=="}, "xaxis": "x", "y": {"dtype": "f8", "bdata": "9oDZA2YP2D9WLrhVLrjlPy5/O8fHSts/NqGesldYwz+EcWIiEo23P76sEqjoLb0/eQPQ5pu2pT+61RmpmFudPw=="}, "yaxis": "y", "type": "scatter"}, {"customdata": [["fact checking"], ["fact checking"], ["fact checking"], ["fact checking"], ["fact checking"], ["fact checking"], ["fact checking"], ["fact checking"]], "hovertemplate": "tarefa=%{customdata[0]}<br>ano=%{x}<br>ocorrências (%)=%{y:.3f}<extra></extra>", "legendgroup": "fact checking", "line": {"dash": "solid"}, "marker": {"symbol": "circle"}, "mode": "lines+markers", "name": "fact checking", "orientation": "v", "showlegend": true, "x": {"dtype": "i2", "bdata": "4QfiB+MH5AflB+YH5wfoBw=="}, "xaxis": "x", "y": {"dtype": "f8", "bdata": "AAAAAAAAAAAAAAAAAAAAAAAAAAAAAAAAAAAAAAAAAAAAAAAAAAAAAClzDHDwc6M/AAAAAAAAAAAAAAAAAAAAAA=="}, "yaxis": "y", "type": "scatter"}, {"customdata": [["forensics"], ["forensics"], ["forensics"], ["forensics"], ["forensics"], ["forensics"], ["forensics"], ["forensics"]], "hovertemplate": "tarefa=%{customdata[0]}<br>ano=%{x}<br>ocorrências (%)=%{y:.3f}<extra></extra>", "legendgroup": "forensics", "line": {"dash": "solid"}, "marker": {"symbol": "circle"}, "mode": "lines+markers", "name": "forensics", "orientation": "v", "showlegend": true, "x": {"dtype": "i2", "bdata": "4QfiB+MH5AflB+YH5wfoBw=="}, "xaxis": "x", "y": {"dtype": "f8", "bdata": "FbFUxFIR6z+Y+iGY+iHYP2OfbNUVeOQ/T9krrAn15D87/O+7niLjP/SPD4zsUNg/WASE4EIkyz8Cm/aTJbDZPw=="}, "yaxis": "y", "type": "scatter"}, {"customdata": [["fraud detection"], ["fraud detection"], ["fraud detection"], ["fraud detection"], ["fraud detection"], ["fraud detection"], ["fraud detection"], ["fraud detection"]], "hovertemplate": "tarefa=%{customdata[0]}<br>ano=%{x}<br>ocorrências (%)=%{y:.3f}<extra></extra>", "legendgroup": "fraud detection", "line": {"dash": "solid"}, "marker": {"symbol": "circle"}, "mode": "lines+markers", "name": "fraud detection", "orientation": "v", "showlegend": true, "x": {"dtype": "i2", "bdata": "4QfiB+MH5AflB+YH5wfoBw=="}, "xaxis": "x", "y": {"dtype": "f8", "bdata": "AAAAAAAAAAAAAAAAAAAAAAAAAAAAAAAAnYHTmB/LqT8AAAAAAAAAAClzDHDwc7M/eQPQ5pu2pT+61RmpmFu9Pw=="}, "yaxis": "y", "type": "scatter"}, {"customdata": [["privacy"], ["privacy"], ["privacy"], ["privacy"], ["privacy"], ["privacy"], ["privacy"], ["privacy"]], "hovertemplate": "tarefa=%{customdata[0]}<br>ano=%{x}<br>ocorrências (%)=%{y:.3f}<extra></extra>", "legendgroup": "privacy", "line": {"dash": "solid"}, "marker": {"symbol": "circle"}, "mode": "lines+markers", "name": "privacy", "orientation": "v", "showlegend": true, "x": {"dtype": "i2", "bdata": "4QfiB+MH5AflB+YH5wfoBw=="}, "xaxis": "x", "y": {"dtype": "f8", "bdata": "9oDZA2YP6D8dk/Uck/XcPxTvIsAgtO4/0PHti4ME7T+XwbYIZe3wP8HIDoWtGfc/IAQXItnI+T+waT9ZApv6Pw=="}, "yaxis": "y", "type": "scatter"}, {"customdata": [["safety"], ["safety"], ["safety"], ["safety"], ["safety"], ["safety"], ["safety"], ["safety"]], "hovertemplate": "tarefa=%{customdata[0]}<br>ano=%{x}<br>ocorrências (%)=%{y:.3f}<extra></extra>", "legendgroup": "safety", "line": {"dash": "solid"}, "marker": {"symbol": "circle"}, "mode": "lines+markers", "name": "safety", "orientation": "v", "showlegend": true, "x": {"dtype": "i2", "bdata": "4QfiB+MH5AflB+YH5wfoBw=="}, "xaxis": "x", "y": {"dtype": "f8", "bdata": "2FBeQ3kN9T8TYk4TYk7zP3Dn+FhpwwJAaRG5pbuR9j+cmIhE4wX5P11Ii+URoQFAjwTxnqx//D/yXTiIHt8EQA=="}, "yaxis": "y", "type": "scatter"}, {"customdata": [["spamming"], ["spamming"], ["spamming"], ["spamming"], ["spamming"], ["spamming"], ["spamming"], ["spamming"]], "hovertemplate": "tarefa=%{customdata[0]}<br>ano=%{x}<br>ocorrências (%)=%{y:.3f}<extra></extra>", "legendgroup": "spamming", "line": {"dash": "solid"}, "marker": {"symbol": "circle"}, "mode": "lines+markers", "name": "spamming", "orientation": "v", "showlegend": true, "x": {"dtype": "i2", "bdata": "4QfiB+MH5AflB+YH5wfoBw=="}, "xaxis": "x", "y": {"dtype": "f8", "bdata": "AAAAAAAAAAAAAAAAAAAAAAAAAAAAAAAAAAAAAAAAAAAAAAAAAAAAAClzDHDwc6M/AAAAAAAAAAC61RmpmFudPw=="}, "yaxis": "y", "type": "scatter"}, {"customdata": [["spoofing"], ["spoofing"], ["spoofing"], ["spoofing"], ["spoofing"], ["spoofing"], ["spoofing"], ["spoofing"]], "hovertemplate": "tarefa=%{customdata[0]}<br>ano=%{x}<br>ocorrências (%)=%{y:.3f}<extra></extra>", "legendgroup": "spoofing", "line": {"dash": "solid"}, "marker": {"symbol": "circle"}, "mode": "lines+markers", "name": "spoofing", "orientation": "v", "showlegend": true, "x": {"dtype": "i2", "bdata": "4QfiB+MH5AflB+YH5wfoBw=="}, "xaxis": "x", "y": {"dtype": "f8", "bdata": "9oDZA2YPuD8TYk4TYk7TP30vhdy8DuE/nYHTmB/L2T8j1cmZzanBP/SPD4zsUMg/eQPQ5pu2xT84iB7fhYPgPw=="}, "yaxis": "y", "type": "scatter"}, {"customdata": [["spotting"], ["spotting"], ["spotting"], ["spotting"], ["spotting"], ["spotting"], ["spotting"], ["spotting"]], "hovertemplate": "tarefa=%{customdata[0]}<br>ano=%{x}<br>ocorrências (%)=%{y:.3f}<extra></extra>", "legendgroup": "spotting", "line": {"dash": "solid"}, "marker": {"symbol": "circle"}, "mode": "lines+markers", "name": "spotting", "orientation": "v", "showlegend": true, "x": {"dtype": "i2", "bdata": "4QfiB+MH5AflB+YH5wfoBw=="}, "xaxis": "x", "y": {"dtype": "f8", "bdata": "9oDZA2YP2D8dk/Uck/XcP2OfbNUVeMQ/0PHti4ME3T8j1cmZzanRP/SPD4zsUMg/eQPQ5pu2xT+UJbBpP1nCPw=="}, "yaxis": "y", "type": "scatter"}], "layout": {"xaxis": {"anchor": "y", "domain": [0.0, 1.0], "title": {"text": "ano"}}, "yaxis": {"anchor": "x", "domain": [0.0, 1.0], "title": {"text": "ocorrências (%)"}}, "legend": {"title": {"text": "tarefa"}, "tracegroupgap": 0}}}
Interpretabilidade e explicabilidade ganharam destaque nos últimos anos, com um aumento significativo no número de artigos publicados sobre o tema por volta de 2019, após a criação de algumas conferências e workshops específicos sobre transparência de modelos, interpretabilidade e equidade, como o ACM FaccT e o VISxAI. A explicabilidade é crucial para construir confiança em sistemas de IA e garantir que suas decisões sejam baseadas em raciocínio válido. Uma das áreas que mais recebeu investimentos nos últimos anos é a fundamentação do modelo, ou seja, o processo de relacionar as previsões do modelo a características específicas dos dados de entrada. Isso é particularmente importante em aplicações como classificação de imagens e resposta a perguntas, onde é essencial compreender quais partes de uma entrada (texto, imagem) estão impulsionando as previsões do modelo.
{"data": [{"customdata": [["explainability"], ["explainability"], ["explainability"], ["explainability"], ["explainability"], ["explainability"], ["explainability"], ["explainability"]], "hovertemplate": "termo=%{customdata[0]}<br>ano=%{x}<br>ocorrências (%)=%{y:.3f}<extra></extra>", "legendgroup": "explainability", "line": {"dash": "solid"}, "marker": {"symbol": "circle"}, "mode": "lines+markers", "name": "explainability", "orientation": "v", "showlegend": true, "x": {"dtype": "i2", "bdata": "4QfiB+MH5AflB+YH5wfoBw=="}, "xaxis": "x", "y": {"dtype": "f8", "bdata": "9oDZA2YPuD8TYk4TYk6zPy5/O8fHSts/AjGEv/Me0D8LrqN3/DDgP76sEqjoLd0/CgP2acj/0j+61RmpmFvdPw=="}, "yaxis": "y", "type": "scatter"}, {"customdata": [["grounding"], ["grounding"], ["grounding"], ["grounding"], ["grounding"], ["grounding"], ["grounding"], ["grounding"]], "hovertemplate": "termo=%{customdata[0]}<br>ano=%{x}<br>ocorrências (%)=%{y:.3f}<extra></extra>", "legendgroup": "grounding", "line": {"dash": "solid"}, "marker": {"symbol": "circle"}, "mode": "lines+markers", "name": "grounding", "orientation": "v", "showlegend": true, "x": {"dtype": "i2", "bdata": "4QfiB+MH5AflB+YH5wfoBw=="}, "xaxis": "x", "y": {"dtype": "f8", "bdata": "uSDjgowL0j9WLrhVLrjlP0kPVM5u4ec/NqGesldY4z+1v65mtH7qP11Ii+URofE/lYMGxlBk9j9ZApv2kyX6Pw=="}, "yaxis": "y", "type": "scatter"}, {"customdata": [["interpretability"], ["interpretability"], ["interpretability"], ["interpretability"], ["interpretability"], ["interpretability"], ["interpretability"], ["interpretability"]], "hovertemplate": "termo=%{customdata[0]}<br>ano=%{x}<br>ocorrências (%)=%{y:.3f}<extra></extra>", "legendgroup": "interpretability", "line": {"dash": "solid"}, "marker": {"symbol": "circle"}, "mode": "lines+markers", "name": "interpretability", "orientation": "v", "showlegend": true, "x": {"dtype": "i2", "bdata": "4QfiB+MH5AflB+YH5wfoBw=="}, "xaxis": "x", "y": {"dtype": "f8", "bdata": "uSDjgowL4j+Y+iGY+iHYP3Dn+Fhpw/I/KQXYtf6J8j+XwbYIZe3wPyM7FLZmnO8/t4JSzKn28D9C9PguHETzPw=="}, "yaxis": "y", "type": "scatter"}, {"customdata": [["traceability"], ["traceability"], ["traceability"], ["traceability"], ["traceability"], ["traceability"], ["traceability"], ["traceability"]], "hovertemplate": "termo=%{customdata[0]}<br>ano=%{x}<br>ocorrências (%)=%{y:.3f}<extra></extra>", "legendgroup": "traceability", "line": {"dash": "solid"}, "marker": {"symbol": "circle"}, "mode": "lines+markers", "name": "traceability", "orientation": "v", "showlegend": true, "x": {"dtype": "i2", "bdata": "4QfiB+MH5AflB+YH5wfoBw=="}, "xaxis": "x", "y": {"dtype": "f8", "bdata": "AAAAAAAAAAAAAAAAAAAAAAAAAAAAAAAAAAAAAAAAAAAAAAAAAAAAAAAAAAAAAAAAAAAAAAAAAAC61RmpmFudPw=="}, "yaxis": "y", "type": "scatter"}], "layout": {"legend": {"title": {"text": "termo"}, "tracegroupgap": 0}, "xaxis": {"anchor": "y", "domain": [0.0, 1.0], "title": {"text": "ano"}}, "yaxis": {"anchor": "x", "domain": [0.0, 1.0], "title": {"text": "ocorrências (%)"}}}}
Tarefas visuais como a remoção de ruído em imagens têm recebido muita atenção nos últimos anos, com muitos artigos publicados sobre o tema. Isso pode ser devido à crescente importância da qualidade das imagens em aplicações de visão computacional, ao desenvolvimento de novas técnicas para aprimorar essa qualidade e à maior capacidade dos modelos visuais de lidar com entradas mais robustas. Essa categoria de tarefas também inclui desembaçamento, remoção de névoa, remoção de moiré, remoção de chuva, entre outras. As tarefas de processamento de imagens e de geração de imagens também aumentaram significativamente.
{"data": [{"customdata": [["colorization"], ["colorization"], ["colorization"], ["colorization"], ["colorization"], ["colorization"], ["colorization"], ["colorization"]], "hovertemplate": "tarefa=%{customdata[0]}<br>ano=%{x}<br>ocorrências (%)=%{y:.3f}<extra></extra>", "legendgroup": "colorization", "line": {"dash": "solid"}, "marker": {"symbol": "circle"}, "mode": "lines+markers", "name": "colorization", "orientation": "v", "showlegend": true, "x": {"dtype": "i2", "bdata": "4QfiB+MH5AflB+YH5wfoBw=="}, "xaxis": "x", "y": {"dtype": "f8", "bdata": "NOHPhD8T3j8dk/Uck/XMPy5/O8fHSts/AjGEv/Me0D+EcWIiEo23P/SPD4zsUMg/eQPQ5pu2tT+UJbBpP1nCPw=="}, "yaxis": "y", "type": "scatter"}, {"customdata": [["denoising"], ["denoising"], ["denoising"], ["denoising"], ["denoising"], ["denoising"], ["denoising"], ["denoising"]], "hovertemplate": "tarefa=%{customdata[0]}<br>ano=%{x}<br>ocorrências (%)=%{y:.3f}<extra></extra>", "legendgroup": "denoising", "line": {"dash": "solid"}, "marker": {"symbol": "circle"}, "mode": "lines+markers", "name": "denoising", "orientation": "v", "showlegend": true, "x": {"dtype": "i2", "bdata": "4QfiB+MH5AflB+YH5wfoBw=="}, "xaxis": "x", "y": {"dtype": "f8", "bdata": "5+ibo2+OBkAdk/Uck/UMQB4lzGKfbA1A6il7hTWhDkAHXUtAUZkLQPSPD4zsUAhAPIRNAY52CkB1RirmVucVQA=="}, "yaxis": "y", "type": "scatter"}, {"customdata": [["editing"], ["editing"], ["editing"], ["editing"], ["editing"], ["editing"], ["editing"], ["editing"]], "hovertemplate": "tarefa=%{customdata[0]}<br>ano=%{x}<br>ocorrências (%)=%{y:.3f}<extra></extra>", "legendgroup": "editing", "line": {"dash": "solid"}, "marker": {"symbol": "circle"}, "mode": "lines+markers", "name": "editing", "orientation": "v", "showlegend": true, "x": {"dtype": "i2", "bdata": "4QfiB+MH5AflB+YH5wfoBw=="}, "xaxis": "x", "y": {"dtype": "f8", "bdata": "9oDZA2YP6D/bxovaxovqP3Dn+Fhpw+I/Qj1lr7Am9D/HDwNNB9/zP4vlEaGp9vs/zYNzhLq/B0CNVMytzkgQQA=="}, "yaxis": "y", "type": "scatter"}, {"customdata": [["image enhancement"], ["image enhancement"], ["image enhancement"], ["image enhancement"], ["image enhancement"], ["image enhancement"], ["image enhancement"], ["image enhancement"]], "hovertemplate": "tarefa=%{customdata[0]}<br>ano=%{x}<br>ocorrências (%)=%{y:.3f}<extra></extra>", "legendgroup": "image enhancement", "line": {"dash": "solid"}, "marker": {"symbol": "circle"}, "mode": "lines+markers", "name": "image enhancement", "orientation": "v", "showlegend": true, "x": {"dtype": "i2", "bdata": "4QfiB+MH5AflB+YH5wfoBw=="}, "xaxis": "x", "y": {"dtype": "f8", "bdata": "AAAAAAAAAACY+iGY+iHYPy5/O8fHSss/nYHTmB/L2T+1v65mtH7aP/SPD4zsUNg/CgP2acj/0j8Vc6szUjHfPw=="}, "yaxis": "y", "type": "scatter"}, {"customdata": [["image filling"], ["image filling"], ["image filling"], ["image filling"], ["image filling"], ["image filling"], ["image filling"], ["image filling"]], "hovertemplate": "tarefa=%{customdata[0]}<br>ano=%{x}<br>ocorrências (%)=%{y:.3f}<extra></extra>", "legendgroup": "image filling", "line": {"dash": "solid"}, "marker": {"symbol": "circle"}, "mode": "lines+markers", "name": "image filling", "orientation": "v", "showlegend": true, "x": {"dtype": "i2", "bdata": "4QfiB+MH5AflB+YH5wfoBw=="}, "xaxis": "x", "y": {"dtype": "f8", "bdata": "JUmSJEmS/D/8rMD7rMD7P4BBaL2RoQBAnYHTmB/LCUD4XU+RqdAGQMDBz028nghAQgNjKDJbBEC1nyyBTfsLQA=="}, "yaxis": "y", "type": "scatter"}, {"customdata": [["image generation"], ["image generation"], ["image generation"], ["image generation"], ["image generation"], ["image generation"], ["image generation"], ["image generation"]], "hovertemplate": "tarefa=%{customdata[0]}<br>ano=%{x}<br>ocorrências (%)=%{y:.3f}<extra></extra>", "legendgroup": "image generation", "line": {"dash": "solid"}, "marker": {"symbol": "circle"}, "mode": "lines+markers", "name": "image generation", "orientation": "v", "showlegend": true, "x": {"dtype": "i2", "bdata": "4QfiB+MH5AflB+YH5wfoBw=="}, "xaxis": "x", "y": {"dtype": "f8", "bdata": "2FBeQ3kN5T8dk/Uck/X8Py5/O8fHSvs/HGkRuaW7AUALrqN3/DAAQIrXkzLHAP8/lYMGxlBkBkC+CwfR47sOQA=="}, "yaxis": "y", "type": "scatter"}, {"customdata": [["image retrieval"], ["image retrieval"], ["image retrieval"], ["image retrieval"], ["image retrieval"], ["image retrieval"], ["image retrieval"], ["image retrieval"]], "hovertemplate": "tarefa=%{customdata[0]}<br>ano=%{x}<br>ocorrências (%)=%{y:.3f}<extra></extra>", "legendgroup": "image retrieval", "line": {"dash": "solid"}, "marker": {"symbol": "circle"}, "mode": "lines+markers", "name": "image retrieval", "orientation": "v", "showlegend": true, "x": {"dtype": "i2", "bdata": "4QfiB+MH5AflB+YH5wfoBw=="}, "xaxis": "x", "y": {"dtype": "f8", "bdata": "uSDjgowL8j9fX19fX1/vP2OfbNUVePQ/XHXyqGLD9T+XwbYIZe3wP8TkCmJyBeE/IAQXItnI6T+UJbBpP1niPw=="}, "yaxis": "y", "type": "scatter"}, {"customdata": [["image segmentation"], ["image segmentation"], ["image segmentation"], ["image segmentation"], ["image segmentation"], ["image segmentation"], ["image segmentation"], ["image segmentation"]], "hovertemplate": "tarefa=%{customdata[0]}<br>ano=%{x}<br>ocorrências (%)=%{y:.3f}<extra></extra>", "legendgroup": "image segmentation", "line": {"dash": "solid"}, "marker": {"symbol": "circle"}, "mode": "lines+markers", "name": "image segmentation", "orientation": "v", "showlegend": true, "x": {"dtype": "i2", "bdata": "4QfiB+MH5AflB+YH5wfoBw=="}, "xaxis": "x", "y": {"dtype": "f8", "bdata": "NOHPhD8T7j81SIM0SIP0P1x7phNsUvU/trlgktFn6z+cmIhE4wXpP/erC2mxPPI/CgP2acj/8j9VzK3OSMX4Pw=="}, "yaxis": "y", "type": "scatter"}, {"customdata": [["image to image"], ["image to image"], ["image to image"], ["image to image"], ["image to image"], ["image to image"], ["image to image"], ["image to image"]], "hovertemplate": "tarefa=%{customdata[0]}<br>ano=%{x}<br>ocorrências (%)=%{y:.3f}<extra></extra>", "legendgroup": "image to image", "line": {"dash": "solid"}, "marker": {"symbol": "circle"}, "mode": "lines+markers", "name": "image to image", "orientation": "v", "showlegend": true, "x": {"dtype": "i2", "bdata": "4QfiB+MH5AflB+YH5wfoBw=="}, "xaxis": "x", "y": {"dtype": "f8", "bdata": "9oDZA2YP2D93FO12FO32P4RTS55mNABAT9krrAn19D8QhXWzekn4P4vlEaGp9us/CgP2acj/4j+n/WQJbNrnPw=="}, "yaxis": "y", "type": "scatter"}, {"customdata": [["localization"], ["localization"], ["localization"], ["localization"], ["localization"], ["localization"], ["localization"], ["localization"]], "hovertemplate": "tarefa=%{customdata[0]}<br>ano=%{x}<br>ocorrências (%)=%{y:.3f}<extra></extra>", "legendgroup": "localization", "line": {"dash": "solid"}, "marker": {"symbol": "circle"}, "mode": "lines+markers", "name": "localization", "orientation": "v", "showlegend": true, "x": {"dtype": "i2", "bdata": "4QfiB+MH5AflB+YH5wfoBw=="}, "xaxis": "x", "y": {"dtype": "f8", "bdata": "2FBeQ3kNFUAD77MC77MSQChbdQUeJQxAaRG5pbuRBkBxIQ48vywOQL2l03D3sg5ASsTocGjNCkC3OiMVc6sMQA=="}, "yaxis": "y", "type": "scatter"}, {"customdata": [["matching"], ["matching"], ["matching"], ["matching"], ["matching"], ["matching"], ["matching"], ["matching"]], "hovertemplate": "tarefa=%{customdata[0]}<br>ano=%{x}<br>ocorrências (%)=%{y:.3f}<extra></extra>", "legendgroup": "matching", "line": {"dash": "solid"}, "marker": {"symbol": "circle"}, "mode": "lines+markers", "name": "matching", "orientation": "v", "showlegend": true, "x": {"dtype": "i2", "bdata": "4QfiB+MH5AflB+YH5wfoBw=="}, "xaxis": "x", "y": {"dtype": "f8", "bdata": "Cn8m/JnwGUCcm5ubm5sTQHJwasnTjBJASYvILd2NFEDbIpS1w/8WQBCM7FDYmhNAV+PLz3ndFEA/WQKb9pMSQA=="}, "yaxis": "y", "type": "scatter"}, {"customdata": [["odometry"], ["odometry"], ["odometry"], ["odometry"], ["odometry"], ["odometry"], ["odometry"], ["odometry"]], "hovertemplate": "tarefa=%{customdata[0]}<br>ano=%{x}<br>ocorrências (%)=%{y:.3f}<extra></extra>", "legendgroup": "odometry", "line": {"dash": "solid"}, "marker": {"symbol": "circle"}, "mode": "lines+markers", "name": "odometry", "orientation": "v", "showlegend": true, "x": {"dtype": "i2", "bdata": "4QfiB+MH5AflB+YH5wfoBw=="}, "xaxis": "x", "y": {"dtype": "f8", "bdata": "9oDZA2YPyD+Y+iGY+iHYP2OfbNUVeNQ/0PHti4ME3T9UIxbeb5vUP/SPD4zsUMg/WASE4EIkyz+UJbBpP1nCPw=="}, "yaxis": "y", "type": "scatter"}, {"customdata": [["quality assessment"], ["quality assessment"], ["quality assessment"], ["quality assessment"], ["quality assessment"], ["quality assessment"], ["quality assessment"], ["quality assessment"]], "hovertemplate": "tarefa=%{customdata[0]}<br>ano=%{x}<br>ocorrências (%)=%{y:.3f}<extra></extra>", "legendgroup": "quality assessment", "line": {"dash": "solid"}, "marker": {"symbol": "circle"}, "mode": "lines+markers", "name": "quality assessment", "orientation": "v", "showlegend": true, "x": {"dtype": "i2", "bdata": "4QfiB+MH5AflB+YH5wfoBw=="}, "xaxis": "x", "y": {"dtype": "f8", "bdata": "9oDZA2YPyD8TYk4TYk7TPy5/O8fHSss/nYHTmB/LyT9UIxbeb5vUP44BDn5u4tU/mwIc7fRI0D84iB7fhYPgPw=="}, "yaxis": "y", "type": "scatter"}, {"customdata": [["reconstruction"], ["reconstruction"], ["reconstruction"], ["reconstruction"], ["reconstruction"], ["reconstruction"], ["reconstruction"], ["reconstruction"]], "hovertemplate": "tarefa=%{customdata[0]}<br>ano=%{x}<br>ocorrências (%)=%{y:.3f}<extra></extra>", "legendgroup": "reconstruction", "line": {"dash": "solid"}, "marker": {"symbol": "circle"}, "mode": "lines+markers", "name": "reconstruction", "orientation": "v", "showlegend": true, "x": {"dtype": "i2", "bdata": "4QfiB+MH5AflB+YH5wfoBw=="}, "xaxis": "x", "y": {"dtype": "f8", "bdata": "vYbyGsprEkDv2p/u2p8WQGOfbNUVeBRAgKIUYNf6F0DN5tSIhfcbQI3zjw+M7BhA3XI9f4LlIUCUJbBpP9keQA=="}, "yaxis": "y", "type": "scatter"}, {"customdata": [["removal"], ["removal"], ["removal"], ["removal"], ["removal"], ["removal"], ["removal"], ["removal"]], "hovertemplate": "tarefa=%{customdata[0]}<br>ano=%{x}<br>ocorrências (%)=%{y:.3f}<extra></extra>", "legendgroup": "removal", "line": {"dash": "solid"}, "marker": {"symbol": "circle"}, "mode": "lines+markers", "name": "removal", "orientation": "v", "showlegend": true, "x": {"dtype": "i2", "bdata": "4QfiB+MH5AflB+YH5wfoBw=="}, "xaxis": "x", "y": {"dtype": "f8", "bdata": "uSDjgowL8j9WLrhVLrj1P0kPVM5u4fc/T9krrAn19D/HDwNNB9/zPyM7FLZmnO8/xwReXRbb7T/mVmekYm7xPw=="}, "yaxis": "y", "type": "scatter"}, {"customdata": [["style transfer"], ["style transfer"], ["style transfer"], ["style transfer"], ["style transfer"], ["style transfer"], ["style transfer"], ["style transfer"]], "hovertemplate": "tarefa=%{customdata[0]}<br>ano=%{x}<br>ocorrências (%)=%{y:.3f}<extra></extra>", "legendgroup": "style transfer", "line": {"dash": "solid"}, "marker": {"symbol": "circle"}, "mode": "lines+markers", "name": "style transfer", "orientation": "v", "showlegend": true, "x": {"dtype": "i2", "bdata": "4QfiB+MH5AflB+YH5wfoBw=="}, "xaxis": "x", "y": {"dtype": "f8", "bdata": "uSDjgowL4j9fX19fX1/vPxTvIsAgtO4/HGkRuaW74T/HDwNNB9/zPylzDHDwc+M/xwReXRbb3T/mVmekYm7hPw=="}, "yaxis": "y", "type": "scatter"}, {"customdata": [["super resolution"], ["super resolution"], ["super resolution"], ["super resolution"], ["super resolution"], ["super resolution"], ["super resolution"], ["super resolution"]], "hovertemplate": "tarefa=%{customdata[0]}<br>ano=%{x}<br>ocorrências (%)=%{y:.3f}<extra></extra>", "legendgroup": "super resolution", "line": {"dash": "solid"}, "marker": {"symbol": "circle"}, "mode": "lines+markers", "name": "super resolution", "orientation": "v", "showlegend": true, "x": {"dtype": "i2", "bdata": "4QfiB+MH5AflB+YH5wfoBw=="}, "xaxis": "x", "y": {"dtype": "f8", "bdata": "2FBeQ3kNBUDrOSbrOSYLQEX9cO2ZTghAsGv9E6UAC0C0/HHkSr4QQFkeEZpqvwpAQgNjKDJbBECwaT9ZApsKQA=="}, "yaxis": "y", "type": "scatter"}], "layout": {"xaxis": {"anchor": "y", "domain": [0.0, 1.0], "title": {"text": "ano"}}, "yaxis": {"anchor": "x", "domain": [0.0, 1.0], "title": {"text": "ocorrências (%)"}}, "legend": {"title": {"text": "tarefa"}, "tracegroupgap": 0}}}
Tarefas de linguagem também têm observado uma variação no número de artigos publicados ao longo dos últimos anos, especialmente aqueles que se concentram em diálogo e conversação. Ao utilizar uma interface conversacional, os usuários podem interagir com sistemas de IA de forma mais natural e intuitiva, proporcionando melhores experiências e uma comunicação mais eficaz. Isso levou a um aumento nas pesquisas sobre sistemas de diálogo, incluindo chatbots, assistentes virtuais e outros agentes conversacionais. O desenvolvimento de modelos de linguagem em larga escala também desempenhou um papel significativo nessa tendência, pois esses modelos demonstraram capacidades impressionantes de gerar textos semelhantes aos humanos e de compreender o contexto.
{"data": [{"customdata": [["dialog"], ["dialog"], ["dialog"], ["dialog"], ["dialog"], ["dialog"], ["dialog"], ["dialog"]], "hovertemplate": "tarefa=%{customdata[0]}<br>ano=%{x}<br>ocorrências (%)=%{y:.3f}<extra></extra>", "legendgroup": "dialog", "line": {"dash": "solid"}, "marker": {"symbol": "circle"}, "mode": "lines+markers", "name": "dialog", "orientation": "v", "showlegend": true, "x": {"dtype": "i2", "bdata": "4QfiB+MH5AflB+YH5wfoBw=="}, "xaxis": "x", "y": {"dtype": "f8", "bdata": "9oDZA2YP2D/RleTQleTgPxTvIsAgtN4/nYHTmB/L2T/lDfuqVnDNPylzDHDwc8M/mwIc7fRIwD+dkYq51RnlPw=="}, "yaxis": "y", "type": "scatter"}, {"customdata": [["language translation"], ["language translation"], ["language translation"], ["language translation"], ["language translation"], ["language translation"], ["language translation"], ["language translation"]], "hovertemplate": "tarefa=%{customdata[0]}<br>ano=%{x}<br>ocorrências (%)=%{y:.3f}<extra></extra>", "legendgroup": "language translation", "line": {"dash": "solid"}, "marker": {"symbol": "circle"}, "mode": "lines+markers", "name": "language translation", "orientation": "v", "showlegend": true, "x": {"dtype": "i2", "bdata": "4QfiB+MH5AflB+YH5wfoBw=="}, "xaxis": "x", "y": {"dtype": "f8", "bdata": "AAAAAAAAAAATYk4TYk6zPwAAAAAAAAAAnYHTmB/LqT+EcWIiEo2nPylzDHDwc7M/eQPQ5pu2pT+61RmpmFudPw=="}, "yaxis": "y", "type": "scatter"}, {"customdata": [["question answering"], ["question answering"], ["question answering"], ["question answering"], ["question answering"], ["question answering"], ["question answering"], ["question answering"]], "hovertemplate": "tarefa=%{customdata[0]}<br>ano=%{x}<br>ocorrências (%)=%{y:.3f}<extra></extra>", "legendgroup": "question answering", "line": {"dash": "solid"}, "marker": {"symbol": "circle"}, "mode": "lines+markers", "name": "question answering", "orientation": "v", "showlegend": true, "x": {"dtype": "i2", "bdata": "4QfiB+MH5AflB+YH5wfoBw=="}, "xaxis": "x", "y": {"dtype": "f8", "bdata": "FbFUxFIR6z8TYk4TYk7zP3Dn+Fhpw+I/nYHTmB/L2T+EcWIiEo3XP/SPD4zsUNg/CgP2acj/4j9C9PguHETjPw=="}, "yaxis": "y", "type": "scatter"}, {"customdata": [["summarization"], ["summarization"], ["summarization"], ["summarization"], ["summarization"], ["summarization"], ["summarization"], ["summarization"]], "hovertemplate": "tarefa=%{customdata[0]}<br>ano=%{x}<br>ocorrências (%)=%{y:.3f}<extra></extra>", "legendgroup": "summarization", "line": {"dash": "solid"}, "marker": {"symbol": "circle"}, "mode": "lines+markers", "name": "summarization", "orientation": "v", "showlegend": true, "x": {"dtype": "i2", "bdata": "4QfiB+MH5AflB+YH5wfoBw=="}, "xaxis": "x", "y": {"dtype": "f8", "bdata": "9oDZA2YP6D+Y+iGY+iHYP2OfbNUVeMQ/nYHTmB/LuT+EcWIiEo23PylzDHDwc8M/eQPQ5pu2tT+UJbBpP1nCPw=="}, "yaxis": "y", "type": "scatter"}, {"customdata": [["text generation"], ["text generation"], ["text generation"], ["text generation"], ["text generation"], ["text generation"], ["text generation"], ["text generation"]], "hovertemplate": "tarefa=%{customdata[0]}<br>ano=%{x}<br>ocorrências (%)=%{y:.3f}<extra></extra>", "legendgroup": "text generation", "line": {"dash": "solid"}, "marker": {"symbol": "circle"}, "mode": "lines+markers", "name": "text generation", "orientation": "v", "showlegend": true, "x": {"dtype": "i2", "bdata": "4QfiB+MH5AflB+YH5wfoBw=="}, "xaxis": "x", "y": {"dtype": "f8", "bdata": "AAAAAAAAAAAAAAAAAAAAAAAAAAAAAAAAAAAAAAAAAACEcWIiEo2nPwAAAAAAAAAAAAAAAAAAAAAAAAAAAAAAAA=="}, "yaxis": "y", "type": "scatter"}], "layout": {"legend": {"title": {"text": "tarefa"}, "tracegroupgap": 0}, "xaxis": {"anchor": "y", "domain": [0.0, 1.0], "title": {"text": "ano"}}, "yaxis": {"anchor": "x", "domain": [0.0, 1.0], "title": {"text": "ocorrências (%)"}}}}
Tarefas multimodais são uma das tendências atuais na inteligência artificial. Essas tarefas envolvem a combinação de diferentes modalidades, como áudio, texto e imagens, para melhorar o desempenho dos modelos e resolver problemas que exigem uma compreensão mais profunda da intermodalidade do mundo. O número de artigos publicados sobre essas tarefas aumentou significativamente nos últimos anos, com um foco especial em tarefas como alinhamento de imagem-texto, síntese de imagens, síntese de vídeos e resposta a perguntas visuais. Essa tendência provavelmente continuará à medida que os pesquisadores exploram novas maneiras de combinar as diferentes modalidades de forma inovadora e aprimoram o desempenho dos modelos.
{"data": [{"customdata": [["alignment"], ["alignment"], ["alignment"], ["alignment"], ["alignment"], ["alignment"], ["alignment"], ["alignment"]], "hovertemplate": "tarefa=%{customdata[0]}<br>ano=%{x}<br>ocorrências (%)=%{y:.3f}<extra></extra>", "legendgroup": "alignment", "line": {"dash": "solid"}, "marker": {"symbol": "circle"}, "mode": "lines+markers", "name": "alignment", "orientation": "v", "showlegend": true, "x": {"dtype": "i2", "bdata": "4QfiB+MH5AflB+YH5wfoBw=="}, "xaxis": "x", "y": {"dtype": "f8", "bdata": "5+ibo2+OBkAD77MC77MCQGCNifRA5QRAiZepHZqVCEAo6V5T4gEQQHYobM04/xJAV+PLz3ndFECuzkjF3OoZQA=="}, "yaxis": "y", "type": "scatter"}, {"customdata": [["audio synthesis"], ["audio synthesis"], ["audio synthesis"], ["audio synthesis"], ["audio synthesis"], ["audio synthesis"], ["audio synthesis"], ["audio synthesis"]], "hovertemplate": "tarefa=%{customdata[0]}<br>ano=%{x}<br>ocorrências (%)=%{y:.3f}<extra></extra>", "legendgroup": "audio synthesis", "line": {"dash": "solid"}, "marker": {"symbol": "circle"}, "mode": "lines+markers", "name": "audio synthesis", "orientation": "v", "showlegend": true, "x": {"dtype": "i2", "bdata": "4QfiB+MH5AflB+YH5wfoBw=="}, "xaxis": "x", "y": {"dtype": "f8", "bdata": "AAAAAAAAAAAAAAAAAAAAAAAAAAAAAAAAAAAAAAAAAAAAAAAAAAAAAClzDHDwc7M/eQPQ5pu2tT+61RmpmFudPw=="}, "yaxis": "y", "type": "scatter"}, {"customdata": [["captioning"], ["captioning"], ["captioning"], ["captioning"], ["captioning"], ["captioning"], ["captioning"], ["captioning"]], "hovertemplate": "tarefa=%{customdata[0]}<br>ano=%{x}<br>ocorrências (%)=%{y:.3f}<extra></extra>", "legendgroup": "captioning", "line": {"dash": "solid"}, "marker": {"symbol": "circle"}, "mode": "lines+markers", "name": "captioning", "orientation": "v", "showlegend": true, "x": {"dtype": "i2", "bdata": "4QfiB+MH5AflB+YH5wfoBw=="}, "xaxis": "x", "y": {"dtype": "f8", "bdata": "JUmSJEmS/D/yexnyexnyP3YLvxoT6fE/T9krrAn15D9UIxbeb5vkP76sEqjoLe0/mwIc7fRI8D/5LhxEj+/2Pw=="}, "yaxis": "y", "type": "scatter"}, {"customdata": [["image synthesis"], ["image synthesis"], ["image synthesis"], ["image synthesis"], ["image synthesis"], ["image synthesis"], ["image synthesis"], ["image synthesis"]], "hovertemplate": "tarefa=%{customdata[0]}<br>ano=%{x}<br>ocorrências (%)=%{y:.3f}<extra></extra>", "legendgroup": "image synthesis", "line": {"dash": "solid"}, "marker": {"symbol": "circle"}, "mode": "lines+markers", "name": "image synthesis", "orientation": "v", "showlegend": true, "x": {"dtype": "i2", "bdata": "4QfiB+MH5AflB+YH5wfoBw=="}, "xaxis": "x", "y": {"dtype": "f8", "bdata": "2FBeQ3kN5T8dk/Uck/X8Py5/O8fHSvs/HGkRuaW7AUALrqN3/DAAQIrXkzLHAP8/lYMGxlBkBkC+CwfR47sOQA=="}, "yaxis": "y", "type": "scatter"}, {"customdata": [["referring expression comprehension"], ["referring expression comprehension"], ["referring expression comprehension"], ["referring expression comprehension"], ["referring expression comprehension"], ["referring expression comprehension"], ["referring expression comprehension"], ["referring expression comprehension"]], "hovertemplate": "tarefa=%{customdata[0]}<br>ano=%{x}<br>ocorrências (%)=%{y:.3f}<extra></extra>", "legendgroup": "referring expression comprehension", "line": {"dash": "solid"}, "marker": {"symbol": "circle"}, "mode": "lines+markers", "name": "referring expression comprehension", "orientation": "v", "showlegend": true, "x": {"dtype": "i2", "bdata": "4QfiB+MH5AflB+YH5wfoBw=="}, "xaxis": "x", "y": {"dtype": "f8", "bdata": "9oDZA2YPuD8TYk4TYk7DPy5/O8fHSrs/NqGesldYwz+EcWIiEo23PylzDHDwc6M/CgP2acj/0j+61RmpmFu9Pw=="}, "yaxis": "y", "type": "scatter"}, {"customdata": [["video question answering"], ["video question answering"], ["video question answering"], ["video question answering"], ["video question answering"], ["video question answering"], ["video question answering"], ["video question answering"]], "hovertemplate": "tarefa=%{customdata[0]}<br>ano=%{x}<br>ocorrências (%)=%{y:.3f}<extra></extra>", "legendgroup": "video question answering", "line": {"dash": "solid"}, "marker": {"symbol": "circle"}, "mode": "lines+markers", "name": "video question answering", "orientation": "v", "showlegend": true, "x": {"dtype": "i2", "bdata": "4QfiB+MH5AflB+YH5wfoBw=="}, "xaxis": "x", "y": {"dtype": "f8", "bdata": "AAAAAAAAAAATYk4TYk6zPy5/O8fHSqs/NqGesldYwz+EcWIiEo3HP/SPD4zsUMg/mwIc7fRI4D9LYNN+sgTWPw=="}, "yaxis": "y", "type": "scatter"}, {"customdata": [["video synthesis"], ["video synthesis"], ["video synthesis"], ["video synthesis"], ["video synthesis"], ["video synthesis"], ["video synthesis"], ["video synthesis"]], "hovertemplate": "tarefa=%{customdata[0]}<br>ano=%{x}<br>ocorrências (%)=%{y:.3f}<extra></extra>", "legendgroup": "video synthesis", "line": {"dash": "solid"}, "marker": {"symbol": "circle"}, "mode": "lines+markers", "name": "video synthesis", "orientation": "v", "showlegend": true, "x": {"dtype": "i2", "bdata": "4QfiB+MH5AflB+YH5wfoBw=="}, "xaxis": "x", "y": {"dtype": "f8", "bdata": "AAAAAAAAAAATYk4TYk7TP0kPVM5u4dc/NqGesldY0z8j1cmZzanRP/SPD4zsUNg/xwReXRbb3T+P78JB9PjwPw=="}, "yaxis": "y", "type": "scatter"}, {"customdata": [["visual grounding"], ["visual grounding"], ["visual grounding"], ["visual grounding"], ["visual grounding"], ["visual grounding"], ["visual grounding"], ["visual grounding"]], "hovertemplate": "tarefa=%{customdata[0]}<br>ano=%{x}<br>ocorrências (%)=%{y:.3f}<extra></extra>", "legendgroup": "visual grounding", "line": {"dash": "solid"}, "marker": {"symbol": "circle"}, "mode": "lines+markers", "name": "visual grounding", "orientation": "v", "showlegend": true, "x": {"dtype": "i2", "bdata": "4QfiB+MH5AflB+YH5wfoBw=="}, "xaxis": "x", "y": {"dtype": "f8", "bdata": "9oDZA2YPuD8dk/Uck/XMPy5/O8fHSrs/nYHTmB/LuT8j1cmZzanRPylzDHDwc9M/CgP2acj/0j9eOIge34XbPw=="}, "yaxis": "y", "type": "scatter"}, {"customdata": [["visual question answering"], ["visual question answering"], ["visual question answering"], ["visual question answering"], ["visual question answering"], ["visual question answering"], ["visual question answering"], ["visual question answering"]], "hovertemplate": "tarefa=%{customdata[0]}<br>ano=%{x}<br>ocorrências (%)=%{y:.3f}<extra></extra>", "legendgroup": "visual question answering", "line": {"dash": "solid"}, "marker": {"symbol": "circle"}, "mode": "lines+markers", "name": "visual question answering", "orientation": "v", "showlegend": true, "x": {"dtype": "i2", "bdata": "4QfiB+MH5AflB+YH5wfoBw=="}, "xaxis": "x", "y": {"dtype": "f8", "bdata": "yLgg44KM8z8+eSo+eSr+P1x7phNsUvU/D81KvEzt8D8LrqN3/DDwP76sEqjoLe0/CgP2acj/8j9QlsCm/WT3Pw=="}, "yaxis": "y", "type": "scatter"}], "layout": {"xaxis": {"anchor": "y", "domain": [0.0, 1.0], "title": {"text": "ano"}}, "yaxis": {"anchor": "x", "domain": [0.0, 1.0], "title": {"text": "ocorrências (%)"}}, "legend": {"title": {"text": "tarefa"}, "tracegroupgap": 0}}}
Aqui nos concentramos em analisar o uso de algumas palavras-chave nos artigos sobre LLM. Mais especificamente:
- Chain-of-Thought, Tree-of-Thought e quaisquer variações de of-Thought - técnicas de prompting que auxiliam o modelo a decompor tarefas complexas em etapas menores e mais gerenciáveis, permitindo que o raciocínio seja realizado de forma mais eficaz;
- Agent - refere-se ao uso de LLMs como agentes que podem executar tarefas de forma autônoma, frequentemente em conjunto com outras ferramentas ou sistemas;
- Distillation - uma técnica utilizada para comprimir modelos grandes em modelos menores e mais eficientes, mantendo seu desempenho;
- Few-shot prompting - uma técnica de prompting que fornece ao modelo alguns exemplos da tarefa em questão, permitindo que ele generalize e execute bem tarefas similares;
- Fine-tuning - o processo de treinar um modelo pré-treinado em uma tarefa ou conjunto de dados específico para melhorar seu desempenho;
- Reinforcement Learning (RL) - um tipo de aprendizagem de máquina onde um agente aprende a tomar decisões recebendo feedback do ambiente na forma de recompensas ou penalidades;
- Retrieval Augmented Generation (RAG) - uma técnica que combina métodos baseados em recuperação com modelos generativos para melhorar o desempenho dos modelos de linguagem em tarefas específicas;
- Self-Instruct - uma técnica que permite que os modelos aprendam com suas próprias saídas, melhorando seu desempenho ao longo do tempo;
- Tokenizer - um componente dos modelos de linguagem que converte o texto em um formato que o modelo possa compreender, frequentemente dividindo-o em unidades menores chamadas tokens;
- Tool - refere-se ao uso de ferramentas ou sistemas externos em conjunto com LLMs para executar tarefas de forma mais eficaz;
- Zero-shot prompting - uma técnica de prompting que permite ao modelo executar tarefas sem exemplos prévios ou treinamento específico para aquela tarefa.
As técnicas de few-shot e zero-shot prompting perderam o interesse da comunidade acadêmica em favor do RAG, dos processos de pensamento (thought) e de novas técnicas de fine-tuning. O interesse em criar agentes LLM capazes de realizar tarefas mais desafiadoras e utilizar ferramentas é um dos tópicos mais quentes na área.
{"data": [{"customdata": [["* of thought"], ["* of thought"], ["* of thought"], ["* of thought"], ["* of thought"], ["* of thought"], ["* of thought"]], "hovertemplate": "termo=%{customdata[0]}<br>ano=%{x}<br>ocorrências (%)=%{y:.3f}<extra></extra>", "legendgroup": "* of thought", "line": {"dash": "solid"}, "marker": {"symbol": "circle"}, "mode": "lines+markers", "name": "* of thought", "orientation": "v", "showlegend": true, "x": {"dtype": "i2", "bdata": "4QfiB+QH5QfmB+cH6Ac="}, "xaxis": "x", "y": {"dtype": "f8", "bdata": "AAAAAAAAAAAAAAAAAAAAAAAAAAAAAAAAAAAAAAAAAAAAAAAAAAAAAAAAAAAAAAAAfDQhL2wVBEA="}, "yaxis": "y", "type": "scatter"}, {"customdata": [["agent"], ["agent"], ["agent"], ["agent"], ["agent"], ["agent"], ["agent"]], "hovertemplate": "termo=%{customdata[0]}<br>ano=%{x}<br>ocorrências (%)=%{y:.3f}<extra></extra>", "legendgroup": "agent", "line": {"dash": "solid"}, "marker": {"symbol": "circle"}, "mode": "lines+markers", "name": "agent", "orientation": "v", "showlegend": true, "x": {"dtype": "i2", "bdata": "4QfiB+QH5QfmB+cH6Ac="}, "xaxis": "x", "y": {"dtype": "f8", "bdata": "AAAAAAAAAAAAAAAAAAAAAAAAAAAAAAAAAAAAAAAAAAAAAAAAAAAAAAAAAAAAAAAABbUZmK5zHEA="}, "yaxis": "y", "type": "scatter"}, {"customdata": [["distillation"], ["distillation"], ["distillation"], ["distillation"], ["distillation"], ["distillation"], ["distillation"]], "hovertemplate": "termo=%{customdata[0]}<br>ano=%{x}<br>ocorrências (%)=%{y:.3f}<extra></extra>", "legendgroup": "distillation", "line": {"dash": "solid"}, "marker": {"symbol": "circle"}, "mode": "lines+markers", "name": "distillation", "orientation": "v", "showlegend": true, "x": {"dtype": "i2", "bdata": "4QfiB+QH5QfmB+cH6Ac="}, "xaxis": "x", "y": {"dtype": "f8", "bdata": "AAAAAAAAAAAAAAAAAAAAAAAAAAAAAAAAAAAAAAAAAAAAAAAAAABJQI7jOI7jOBZAus6xRiIgDkA="}, "yaxis": "y", "type": "scatter"}, {"customdata": [["few shot"], ["few shot"], ["few shot"], ["few shot"], ["few shot"], ["few shot"], ["few shot"]], "hovertemplate": "termo=%{customdata[0]}<br>ano=%{x}<br>ocorrências (%)=%{y:.3f}<extra></extra>", "legendgroup": "few shot", "line": {"dash": "solid"}, "marker": {"symbol": "circle"}, "mode": "lines+markers", "name": "few shot", "orientation": "v", "showlegend": true, "x": {"dtype": "i2", "bdata": "4QfiB+QH5QfmB+cH6Ac="}, "xaxis": "x", "y": {"dtype": "f8", "bdata": "AAAAAAAAAAAAAAAAAAAAAAAAAAAAAAAAAAAAAAAAAAAAAAAAAAAAAKuqqqqqqjBAMU653d/BJUA="}, "yaxis": "y", "type": "scatter"}, {"customdata": [["finetuning"], ["finetuning"], ["finetuning"], ["finetuning"], ["finetuning"], ["finetuning"], ["finetuning"]], "hovertemplate": "termo=%{customdata[0]}<br>ano=%{x}<br>ocorrências (%)=%{y:.3f}<extra></extra>", "legendgroup": "finetuning", "line": {"dash": "solid"}, "marker": {"symbol": "circle"}, "mode": "lines+markers", "name": "finetuning", "orientation": "v", "showlegend": true, "x": {"dtype": "i2", "bdata": "4QfiB+QH5QfmB+cH6Ac="}, "xaxis": "x", "y": {"dtype": "f8", "bdata": "AAAAAAAAAAAAAAAAAAAAAAAAAAAAAAAAAAAAAAAAAAAAAAAAAAAAAI7jOI7jOCZAus6xRiIgLkA="}, "yaxis": "y", "type": "scatter"}, {"customdata": [["reinforcement learning"], ["reinforcement learning"], ["reinforcement learning"], ["reinforcement learning"], ["reinforcement learning"], ["reinforcement learning"], ["reinforcement learning"]], "hovertemplate": "termo=%{customdata[0]}<br>ano=%{x}<br>ocorrências (%)=%{y:.3f}<extra></extra>", "legendgroup": "reinforcement learning", "line": {"dash": "solid"}, "marker": {"symbol": "circle"}, "mode": "lines+markers", "name": "reinforcement learning", "orientation": "v", "showlegend": true, "x": {"dtype": "i2", "bdata": "4QfiB+QH5QfmB+cH6Ac="}, "xaxis": "x", "y": {"dtype": "f8", "bdata": "AAAAAAAASUAAAAAAAAAAAAAAAAAAAAAAAAAAAAAAAAAAAAAAAAAAAKuqqqqqqjBA3P0dXPaGQEA="}, "yaxis": "y", "type": "scatter"}, {"customdata": [["retrieval augmented generation"], ["retrieval augmented generation"], ["retrieval augmented generation"], ["retrieval augmented generation"], ["retrieval augmented generation"], ["retrieval augmented generation"], ["retrieval augmented generation"]], "hovertemplate": "termo=%{customdata[0]}<br>ano=%{x}<br>ocorrências (%)=%{y:.3f}<extra></extra>", "legendgroup": "retrieval augmented generation", "line": {"dash": "solid"}, "marker": {"symbol": "circle"}, "mode": "lines+markers", "name": "retrieval augmented generation", "orientation": "v", "showlegend": true, "x": {"dtype": "i2", "bdata": "4QfiB+QH5QfmB+cH6Ac="}, "xaxis": "x", "y": {"dtype": "f8", "bdata": "AAAAAAAAOUAAAAAAAAAAAAAAAAAAAAAAAAAAAAAAAAAAAAAAAABJQKuqqqqqqkBAayQC4qMJQ0A="}, "yaxis": "y", "type": "scatter"}, {"customdata": [["tokenizer"], ["tokenizer"], ["tokenizer"], ["tokenizer"], ["tokenizer"], ["tokenizer"], ["tokenizer"]], "hovertemplate": "termo=%{customdata[0]}<br>ano=%{x}<br>ocorrências (%)=%{y:.3f}<extra></extra>", "legendgroup": "tokenizer", "line": {"dash": "solid"}, "marker": {"symbol": "circle"}, "mode": "lines+markers", "name": "tokenizer", "orientation": "v", "showlegend": true, "x": {"dtype": "i2", "bdata": "4QfiB+QH5QfmB+cH6Ac="}, "xaxis": "x", "y": {"dtype": "f8", "bdata": "AAAAAAAAAAAAAAAAAAAAAAAAAAAAAAAAAAAAAAAAAAAAAAAAAAAAAAAAAAAAAAAAfDQhL2wV9D8="}, "yaxis": "y", "type": "scatter"}, {"customdata": [["tool"], ["tool"], ["tool"], ["tool"], ["tool"], ["tool"], ["tool"]], "hovertemplate": "termo=%{customdata[0]}<br>ano=%{x}<br>ocorrências (%)=%{y:.3f}<extra></extra>", "legendgroup": "tool", "line": {"dash": "solid"}, "marker": {"symbol": "circle"}, "mode": "lines+markers", "name": "tool", "orientation": "v", "showlegend": true, "x": {"dtype": "i2", "bdata": "4QfiB+QH5QfmB+cH6Ac="}, "xaxis": "x", "y": {"dtype": "f8", "bdata": "AAAAAAAAOUAAAAAAAAAAAAAAAAAAAElAAAAAAAAAAAAAAAAAAAAAAAAAAAAAAAAAMU653d/BFUA="}, "yaxis": "y", "type": "scatter"}, {"customdata": [["zero shot"], ["zero shot"], ["zero shot"], ["zero shot"], ["zero shot"], ["zero shot"], ["zero shot"]], "hovertemplate": "termo=%{customdata[0]}<br>ano=%{x}<br>ocorrências (%)=%{y:.3f}<extra></extra>", "legendgroup": "zero shot", "line": {"dash": "solid"}, "marker": {"symbol": "circle"}, "mode": "lines+markers", "name": "zero shot", "orientation": "v", "showlegend": true, "x": {"dtype": "i2", "bdata": "4QfiB+QH5QfmB+cH6Ac="}, "xaxis": "x", "y": {"dtype": "f8", "bdata": "AAAAAAAAAAAAAAAAAAAAAAAAAAAAAAAAAAAAAAAAAAAAAAAAAABJQBzHcRzHcUNAoifVVzI/M0A="}, "yaxis": "y", "type": "scatter"}], "layout": {"legend": {"title": {"text": "termo"}, "tracegroupgap": 0}, "xaxis": {"anchor": "y", "domain": [0.0, 1.0], "title": {"text": "ano"}}, "yaxis": {"anchor": "x", "domain": [0.0, 1.0], "title": {"text": "ocorrências (%)"}}}}
Informações sobre os Autores
Agora, vamos analisar os autores dos artigos. Este primeiro gráfico mostra o número de artigos publicados por cada autor. Como podemos ver, a maioria dos autores publicou apenas um artigo na conferência. De um total de 33.861 autores, somente 1.308 possuem 10 ou mais artigos aceitos.
{"data": [{"hovertemplate": "Número de artigos=%{text}<br>Autores=%{y}<extra></extra>", "legendgroup": "", "marker": {"pattern": {"shape": ""}}, "name": "", "orientation": "v", "showlegend": false, "text": {"dtype": "f8", "bdata": "AAAAAADAYEAAAAAAAMBdQAAAAAAAAFlAAAAAAACAVUAAAAAAAMBUQAAAAAAAAFRAAAAAAADAU0AAAAAAAMBSQAAAAAAAwFFAAAAAAACAUUAAAAAAAEBRQAAAAAAAgFBAAAAAAABAUEAAAAAAAABQQAAAAAAAgE9AAAAAAAAAT0AAAAAAAIBOQAAAAAAAgE1AAAAAAAAATUAAAAAAAABMQAAAAAAAgEtAAAAAAAAAS0AAAAAAAIBKQAAAAAAAAEpAAAAAAACASUAAAAAAAABJQAAAAAAAgEhAAAAAAAAASEAAAAAAAIBHQAAAAAAAAEdAAAAAAAAARkAAAAAAAIBFQAAAAAAAAEVAAAAAAACAREAAAAAAAABEQAAAAAAAgENAAAAAAAAAQ0AAAAAAAIBCQAAAAAAAAEJAAAAAAACAQUAAAAAAAABBQAAAAAAAgEBAAAAAAAAAQEAAAAAAAAA/QAAAAAAAAD5AAAAAAAAAPUAAAAAAAAA8QAAAAAAAADtAAAAAAAAAOkAAAAAAAAA5QAAAAAAAADhAAAAAAAAAN0AAAAAAAAA2QAAAAAAAADVAAAAAAAAANEAAAAAAAAAzQAAAAAAAADJAAAAAAAAAMUAAAAAAAAAwQAAAAAAAAC5AAAAAAAAALEAAAAAAAAAqQAAAAAAAAChAAAAAAAAAJkAAAAAAAAAkQAAAAAAAACJAAAAAAAAAIEAAAAAAAAAcQAAAAAAAABhAAAAAAAAAFEAAAAAAAAAQQAAAAAAAAAhAAAAAAAAAAEAAAAAAAADwPw=="}, "textposition": "auto", "x": {"dtype": "i2", "bdata": "hgB3AGQAVgBTAFAATwBLAEcARgBFAEIAQQBAAD8APgA9ADsAOgA4ADcANgA1ADQAMwAyADEAMAAvAC4ALAArACoAKQAoACcAJgAlACQAIwAiACEAIAAfAB4AHQAcABsAGgAZABgAFwAWABUAFAATABIAEQAQAA8ADgANAAwACwAKAAkACAAHAAYABQAEAAMAAgABAA=="}, "xaxis": "x", "y": {"dtype": "i2", "bdata": "AQABAAEAAQABAAEAAQABAAEAAQABAAEAAQABAAIAAQABAAEABQAEAAIAAQABAAMAAQACAAIABAABAAIABAAEAAQAAgAHAAgACwAGAAsABAAFAAYAFAALABIACwASABEAEgAVAB4AGAAWACQAIgAyACMALABAAFEAWABrAHsAlgCzAAIBKgGeAUMCSQOXBW8J3RU8UQ=="}, "yaxis": "y", "type": "bar"}], "layout": {"xaxis": {"anchor": "y", "domain": [0.0, 1.0], "title": {"text": "Número de artigos"}}, "yaxis": {"anchor": "x", "domain": [0.0, 1.0], "title": {"text": "Autores"}}, "legend": {"tracegroupgap": 0}, "barmode": "relative"}}
Aqui estão os 10 autores com mais artigos:
Autor | Artigos |
---|---|
Luc Van Gool | 134 |
Radu Timofte | 119 |
Lei Zhang | 100 |
Yi Yang | 86 |
Yu Qiao | 83 |
Dacheng Tao | 80 |
Ming-Hsuan Yang | 79 |
Qi Tian | 75 |
Marc Pollefeys | 71 |
Xiaogang Wang | 70 |
Agora vamos analisar o número de autores por artigo. A maioria dos artigos tem entre 2 e 7 autores, mas há alguns com um número elevado de autores, como Why Is the Winner the Best?, que conta com 125 autores, e The Ninth NTIRE 2024 Efficient Super-Resolution Challenge Report, com impressionantes 134 autores. O primeiro é um estudo multicêntrico de todas as 80 competições realizadas no âmbito do IEEE ISBI 2021 e MICCAI 2021, enquanto o segundo é um relatório que resume os resultados do desafio NTIRE 2024, uma competição realizada na conferência CVPR.
{"data": [{"hovertemplate": "Número de autores=%{x}<br>Número de artigos=%{text}<extra></extra>", "legendgroup": "", "marker": {"pattern": {"shape": ""}}, "name": "", "orientation": "v", "showlegend": false, "text": {"dtype": "f8", "bdata": "AAAAAAAgZ0AAAAAAAByWQAAAAAAAoqZAAAAAAAAArEAAAAAAAE6pQAAAAAAA2KJAAAAAAADklkAAAAAAALiIQAAAAAAA0HhAAAAAAADAaUAAAAAAAIBcQAAAAAAAAFBAAAAAAAAAOUAAAAAAAAA3QAAAAAAAACRAAAAAAAAAJkAAAAAAAAAQQAAAAAAAAABAAAAAAAAA8D8AAAAAAAAQQAAAAAAAAABAAAAAAAAAAEAAAAAAAAAQQAAAAAAAAAhAAAAAAAAA8D8AAAAAAAAIQAAAAAAAAPA/AAAAAAAA8D8AAAAAAADwPwAAAAAAAPA/AAAAAAAAAEAAAAAAAAAAQAAAAAAAAPA/AAAAAAAA8D8AAAAAAAAAQAAAAAAAAPA/AAAAAAAA8D8AAAAAAADwPwAAAAAAAPA/AAAAAAAA8D8AAAAAAADwPwAAAAAAAPA/AAAAAAAACEAAAAAAAADwPwAAAAAAAPA/AAAAAAAACEAAAAAAAADwPwAAAAAAAPA/AAAAAAAA8D8AAAAAAADwPwAAAAAAAPA/AAAAAAAA8D8AAAAAAADwPwAAAAAAAPA/AAAAAAAA8D8AAAAAAADwPwAAAAAAAPA/AAAAAAAA8D8="}, "textposition": "auto", "x": {"dtype": "i2", "bdata": "AQACAAMABAAFAAYABwAIAAkACgALAAwADQAOAA8AEAARABIAEwAUABUAFgAXABgAGwAcAB8AIQAiACMAJAAlACcAKQAqACsALQAwADEANQA3ADgAOgBCAEMARABOAE8AVQBYAF0AZABlAGwAcQBzAH0AhgA="}, "xaxis": "x", "y": {"dtype": "i2", "bdata": "uQCHBVELAA6nDGwJuQUXA40BzgByAEAAGQAXAAoACwAEAAIAAQAEAAIAAgAEAAMAAQADAAEAAQABAAEAAgACAAEAAQACAAEAAQABAAEAAQABAAEAAwABAAEAAwABAAEAAQABAAEAAQABAAEAAQABAAEAAQA="}, "yaxis": "y", "type": "bar"}], "layout": {"xaxis": {"anchor": "y", "domain": [0.0, 1.0], "title": {"text": "Número de autores"}}, "yaxis": {"anchor": "x", "domain": [0.0, 1.0], "title": {"text": "Número de artigos"}}, "legend": {"tracegroupgap": 0}, "barmode": "relative"}}
Como a maioria dos artigos tem múltiplos autores, é bastante comum ver alguns autores colaborando constantemente entre si. O par de autores mais comum é Jiwen Lu e Jie Zhou, que colaboraram em 57 artigos juntos. O segundo par mais comum é Luc Van Gool e Radu Timofte, com 43 artigos juntos, seguido por Tao Xiang e Yi-Zhe Song, com 38 artigos. Os 10 pares de autores mais frequentes são:
Autor 1 | Autor 2 | Artigos |
---|---|---|
Jiwen Lu | Jie Zhou | 57 |
Luc Van Gool | Radu Timofte | 43 |
Tao Xiang | Yi-Zhe Song | 38 |
Fahad Shahbaz Khan | Salman Khan | 33 |
Ting Yao | Tao Mei | 32 |
Xiaogang Wang | Hongsheng Li | 28 |
Shiguang Shan | Xilin Chen | 27 |
Richa Singh | Mayank Vatsa | 26 |
Dong Chen | Fang Wen | 24 |
Yi-Zhe Song | Ayan Kumar Bhunia | 24 |
Embora seja bastante raro que um artigo tenha um único autor, 185 trabalhos se enquadram nessa categoria. Algumas menções que merecem destaque incluem pesquisas que introduziram funções de perda inovadoras (Jonathan T. Barron, Takumi Kobayashi) e aprimoramentos em arquiteturas de transformers e técnicas de pós-treinamento (Takumi Kobayashi, Jing Ma). Nesta tabela, podemos ver os autores com o maior número de artigos nos quais são os únicos autores.
Autor | Artigos |
---|---|
Takumi Kobayashi | 4 |
Anant Khandelwal, Takuhiro Kaneko | 3 |
Andrey V. Savchenko, Chong Yu, Dimitrios Kollias, Edgar A. Bernal, Jamie Hayes, Magnus Oskarsson, Ming Li, Oleksii Sidorov, Ren Yang, Rowel Atienza, Sanghwa Hong, Satoshi Ikehata, Shunta Maeda, Stamatios Lefkimmiatis, Ying Zhao | 2 |
Identificando Tópicos
Para esta seção, utilizamos o Top2Vec, um algoritmo de modelagem automática de tópicos, para identificar grupos de artigos que são semelhantes entre si com base em seus títulos e resumos. A solução identificou 172 tópicos, o que é um número um pouco alto para analisarmos individualmente. Em vez disso, focaremos nos tópicos mais quentes e mais frios, ou seja, aqueles com o maior e o menor número de artigos no último ano, respectivamente.
Um problema com o algoritmo é que ele identifica tópicos com base nas palavras utilizadas nos artigos, mas não fornece uma explicação clara sobre o que esses tópicos abordam. Esse é um desafio comum em algoritmos de modelagem de tópicos, que frequentemente produzem resultados difíceis de interpretar. No entanto, podemos utilizar LLMs para nos ajudar a compreender o significado desses tópicos. Usaremos as palavras mais representativas de cada tópico (aquelas que aparecem com maior frequência nos artigos daquele tópico) para gerar um título e um parágrafo que o resuma.
🔥 10 tópicos
{"data": [{"customdata": {"dtype": "i1", "bdata": "AQEBAQEBAQE=", "shape": "8, 1"}, "hovertemplate": "tópico=%{customdata[0]}<br>ano=%{x}<br>ocorrências (%)=%{y:.3f}<extra></extra>", "legendgroup": "1", "line": {"dash": "solid"}, "marker": {"symbol": "circle"}, "mode": "lines+markers", "name": "1", "orientation": "v", "showlegend": true, "x": {"dtype": "i2", "bdata": "4QfiB+MH5AflB+YH5wfoBw=="}, "xaxis": "x", "y": {"dtype": "f8", "bdata": "AAAAAAAAAAATYk4TYk6zPy5/O8fHSrs/nYHTmB/LqT8AAAAAAAAAAClzDHDwc6M/WASE4EIk2z+NVMytzkgQQA=="}, "yaxis": "y", "type": "scatter"}, {"customdata": {"dtype": "i1", "bdata": "AgICAgICAgI=", "shape": "8, 1"}, "hovertemplate": "tópico=%{customdata[0]}<br>ano=%{x}<br>ocorrências (%)=%{y:.3f}<extra></extra>", "legendgroup": "2", "line": {"dash": "solid"}, "marker": {"symbol": "circle"}, "mode": "lines+markers", "name": "2", "orientation": "v", "showlegend": true, "x": {"dtype": "i2", "bdata": "4QfiB+MH5AflB+YH5wfoBw=="}, "xaxis": "x", "y": {"dtype": "f8", "bdata": "9oDZA2YP2D9WLrhVLrjlPy5/O8fHSus/T9krrAn19D/gNilv2Ff1P1keEZpqv/o/IAQXItnICUBXZ6RibnUZQA=="}, "yaxis": "y", "type": "scatter"}, {"customdata": {"dtype": "i1", "bdata": "AwMDAwMDAwM=", "shape": "8, 1"}, "hovertemplate": "tópico=%{customdata[0]}<br>ano=%{x}<br>ocorrências (%)=%{y:.3f}<extra></extra>", "legendgroup": "3", "line": {"dash": "solid"}, "marker": {"symbol": "circle"}, "mode": "lines+markers", "name": "3", "orientation": "v", "showlegend": true, "x": {"dtype": "i2", "bdata": "4QfiB+MH5AflB+YH5wfoBw=="}, "xaxis": "x", "y": {"dtype": "f8", "bdata": "yLgg44KMA0DhCH/hCH8BQHodovvnewFAHGkRuaW7AUD4XU+RqdD2PyhljgEOfvY/XoOZB+cI9T/+ZAls2k8IQA=="}, "yaxis": "y", "type": "scatter"}, {"customdata": {"dtype": "i1", "bdata": "BAQEBAQEBAQ=", "shape": "8, 1"}, "hovertemplate": "tópico=%{customdata[0]}<br>ano=%{x}<br>ocorrências (%)=%{y:.3f}<extra></extra>", "legendgroup": "4", "line": {"dash": "solid"}, "marker": {"symbol": "circle"}, "mode": "lines+markers", "name": "4", "orientation": "v", "showlegend": true, "x": {"dtype": "i2", "bdata": "4QfiB+MH5AflB+YH5wfoBw=="}, "xaxis": "x", "y": {"dtype": "f8", "bdata": "AAAAAAAAAAATYk4TYk6zPy5/O8fHSrs/nYHTmB/LqT8AAAAAAAAAAClzDHDwc7M/CgP2acj/0j+rM1Ixtzr5Pw=="}, "yaxis": "y", "type": "scatter"}, {"customdata": {"dtype": "i1", "bdata": "BQUFBQUFBQU=", "shape": "8, 1"}, "hovertemplate": "tópico=%{customdata[0]}<br>ano=%{x}<br>ocorrências (%)=%{y:.3f}<extra></extra>", "legendgroup": "5", "line": {"dash": "solid"}, "marker": {"symbol": "circle"}, "mode": "lines+markers", "name": "5", "orientation": "v", "showlegend": true, "x": {"dtype": "i2", "bdata": "4QfiB+MH5AflB+YH5wfoBw=="}, "xaxis": "x", "y": {"dtype": "f8", "bdata": "qYilIpaKAEBWLrhVLrgFQFZX4FHCLAZAL1M7NCvxAkCcmIhE4wX5P43zjw+M7Pg/mwIc7fRI4D8MB9Hju3D8Pw=="}, "yaxis": "y", "type": "scatter"}, {"customdata": {"dtype": "i1", "bdata": "BgYGBgYGBgY=", "shape": "8, 1"}, "hovertemplate": "tópico=%{customdata[0]}<br>ano=%{x}<br>ocorrências (%)=%{y:.3f}<extra></extra>", "legendgroup": "6", "line": {"dash": "solid"}, "marker": {"symbol": "circle"}, "mode": "lines+markers", "name": "6", "orientation": "v", "showlegend": true, "x": {"dtype": "i2", "bdata": "4QfiB+MH5AflB+YH5wfoBw=="}, "xaxis": "x", "y": {"dtype": "f8", "bdata": "NOHPhD8T3j9WLrhVLrjlP0kPVM5u4ec/0PHti4ME3T87/O+7niLjP1keEZpqv9o/eQPQ5pu2tT9VzK3OSMXoPw=="}, "yaxis": "y", "type": "scatter"}, {"customdata": {"dtype": "i1", "bdata": "BwcHBwcHBwc=", "shape": "8, 1"}, "hovertemplate": "tópico=%{customdata[0]}<br>ano=%{x}<br>ocorrências (%)=%{y:.3f}<extra></extra>", "legendgroup": "7", "line": {"dash": "solid"}, "marker": {"symbol": "circle"}, "mode": "lines+markers", "name": "7", "orientation": "v", "showlegend": true, "x": {"dtype": "i2", "bdata": "4QfiB+MH5AflB+YH5wfoBw=="}, "xaxis": "x", "y": {"dtype": "f8", "bdata": "9oDZA2YPuD8AAAAAAAAAAAAAAAAAAAAAnYHTmB/LuT+EcWIiEo2nPylzDHDwc8M/0wKJq16k4T+ZW52RirnzPw=="}, "yaxis": "y", "type": "scatter"}, {"customdata": {"dtype": "i1", "bdata": "CAgICAgICAg=", "shape": "8, 1"}, "hovertemplate": "tópico=%{customdata[0]}<br>ano=%{x}<br>ocorrências (%)=%{y:.3f}<extra></extra>", "legendgroup": "8", "line": {"dash": "solid"}, "marker": {"symbol": "circle"}, "mode": "lines+markers", "name": "8", "orientation": "v", "showlegend": true, "x": {"dtype": "i2", "bdata": "4QfiB+MH5AflB+YH5wfoBw=="}, "xaxis": "x", "y": {"dtype": "f8", "bdata": "uSDjgowL4j9WLrhVLrjlPy5/O8fHSqs/NqGesldYwz+EcWIiEo3HPyM7FLZmnN8/AAAAAAAAAADwwkH0+C7kPw=="}, "yaxis": "y", "type": "scatter"}, {"customdata": {"dtype": "i1", "bdata": "CQkJCQkJCQk=", "shape": "8, 1"}, "hovertemplate": "tópico=%{customdata[0]}<br>ano=%{x}<br>ocorrências (%)=%{y:.3f}<extra></extra>", "legendgroup": "9", "line": {"dash": "solid"}, "marker": {"symbol": "circle"}, "mode": "lines+markers", "name": "9", "orientation": "v", "showlegend": true, "x": {"dtype": "i2", "bdata": "4QfiB+MH5AflB+YH5wfoBw=="}, "xaxis": "x", "y": {"dtype": "f8", "bdata": "NOHPhD8T/j9fX19fX1/vP3Dn+Fhpw+I/nYHTmB/L6T8j1cmZzanhP1keEZpqv9o/AAAAAAAAAACUJbBpP1niPw=="}, "yaxis": "y", "type": "scatter"}, {"customdata": {"dtype": "i1", "bdata": "CgoKCgoKCgo=", "shape": "8, 1"}, "hovertemplate": "tópico=%{customdata[0]}<br>ano=%{x}<br>ocorrências (%)=%{y:.3f}<extra></extra>", "legendgroup": "10", "line": {"dash": "solid"}, "marker": {"symbol": "circle"}, "mode": "lines+markers", "name": "10", "orientation": "v", "showlegend": true, "x": {"dtype": "i2", "bdata": "4QfiB+MH5AflB+YH5wfoBw=="}, "xaxis": "x", "y": {"dtype": "f8", "bdata": "uSDjgowL4j/RleTQleTgP2nDMpe/nfM/0PHti4ME3T+1v65mtH7qP/erC2mxPOI/0wKJq16k4T/mVmekYm7xPw=="}, "yaxis": "y", "type": "scatter"}], "layout": {"legend": {"title": {"text": "tópico"}, "tracegroupgap": 0}, "xaxis": {"anchor": "y", "domain": [0.0, 1.0], "title": {"text": "ano"}}, "yaxis": {"anchor": "x", "domain": [0.0, 1.0], "title": {"text": "ocorrências (%)"}}}}
Os tópicos abaixo estão listados na ordem em que tiveram mais artigos publicados no ano passado.
Tópico 1 - Instruction-Tuned Multimodal LLMs for Vision-Language Understanding (157 documents)
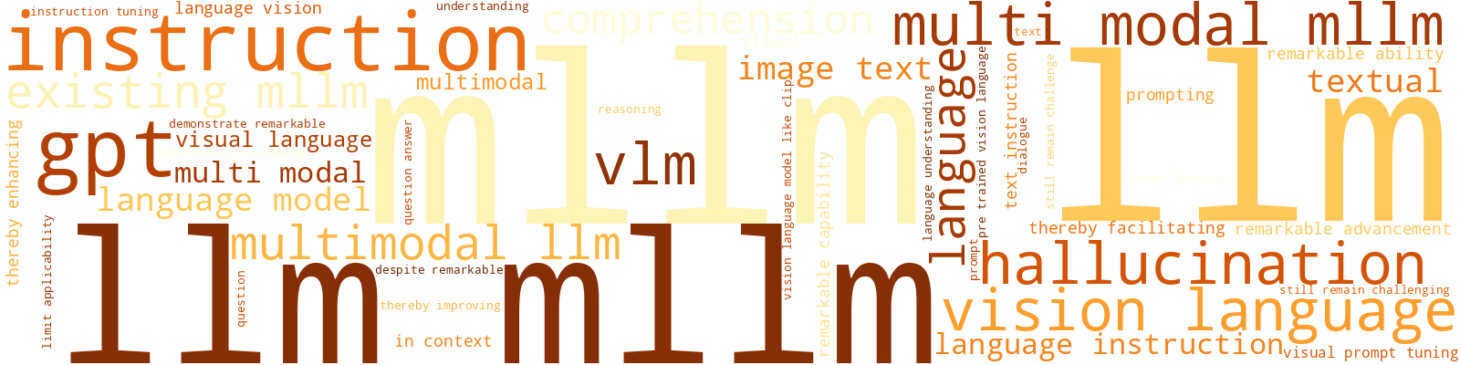
Principais autores:
- Yu Qiao (7 artigos)
- Ying Shan (5 artigos)
- Yixiao Ge (5 artigos)
- LLM-Seg: Bridging Image Segmentation and Large Language Model Reasoning (2024)
- HallusionBench: An Advanced Diagnostic Suite for Entangled Language Hallucination and Visual Illusion in Large Vision-Language Models (2024)
- Rugby Scene Classification Enhanced by Vision Language Model (2024)
Tópico 2 - Controllable Text-Guided Image Editing with Diffusion and GAN Inversion (426 documents)
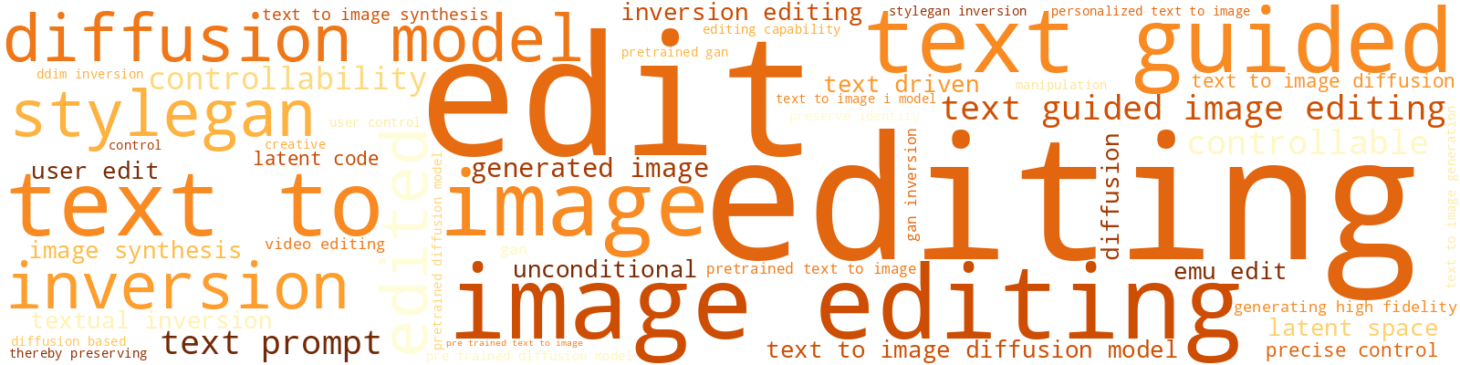
Principais autores:
- Chen Change Loy (9 artigos)
- Xintao Wang (8 artigos)
- Ying Shan (8 artigos)
- iEdit: Localised Text-guided Image Editing with Weak Supervision (2024)
- MoMask: Generative Masked Modeling of 3D Human Motions (2024)
- Contrastive Denoising Score for Text-guided Latent Diffusion Image Editing (2024)
Tópico 3 - AI-Driven Medical Imaging and Diagnosis in Clinical Practice (345 documents)
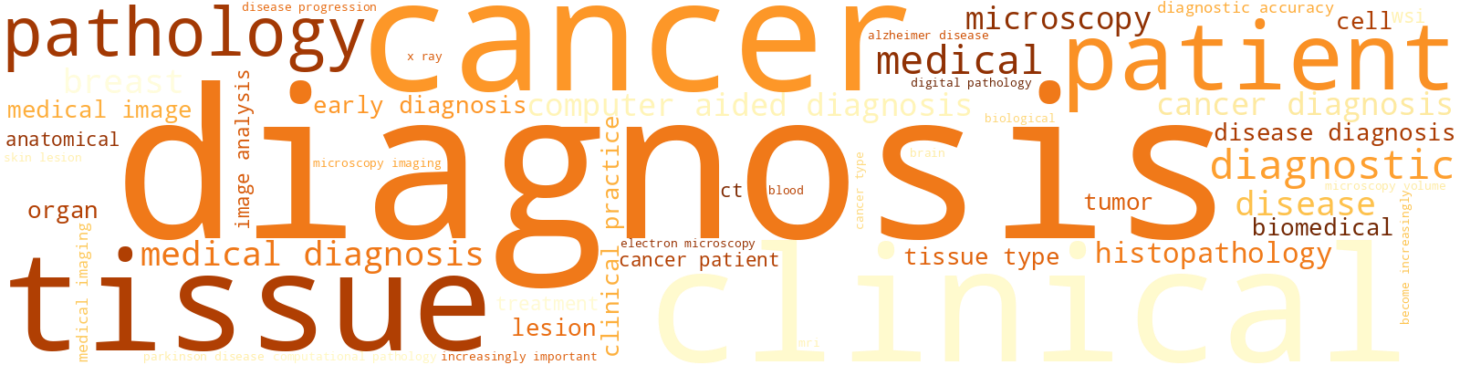
Principais autores:
- Le Lu (9 artigos)
- Faisal Mahmood (5 artigos)
- Ke Yan (5 artigos)
- SQUID: Deep Feature In-Painting for Unsupervised Anomaly Detection (2023)
- A Logarithmic X-Ray Imaging Model for Baggage Inspection: Simulation and Object Detection (2017)
- DiRA: Discriminative, Restorative, and Adversarial Learning for Self-Supervised Medical Image Analysis (2022)
Tópico 4 - Challenges and Advances in 3D-Aware Text-to-Image and Text-to-Video Generation (68 documents)
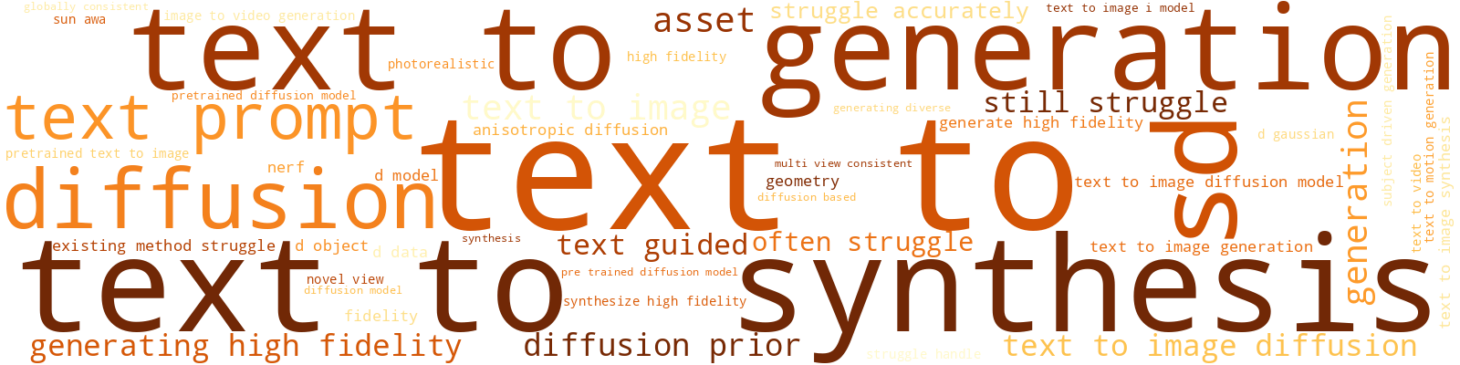
Principais autores:
- Hsin-Ying Lee (4 artigos)
- Sergey Tulyakov (4 artigos)
- Ying Shan (4 artigos)
- Enhancing 3D Fidelity of Text-to-3D using Cross-View Correspondences (2024)
- DIRECT-3D: Learning Direct Text-to-3D Generation on Massive Noisy 3D Data (2024)
- Diffusion Time-step Curriculum for One Image to 3D Generation (2024)
Tópico 5 - Remote Sensing and Aerial Imagery for Environmental and Agricultural Monitoring (306 documents)
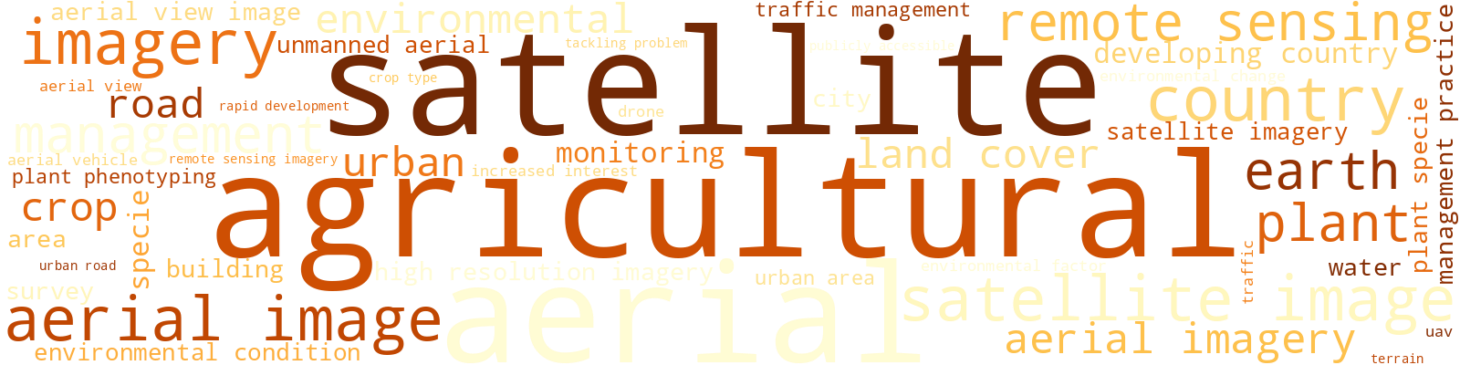
Principais autores:
- Sara Beery (5 artigos)
- David Lobell (4 artigos)
- Edward J. Delp (4 artigos)
- Unsupervised Learning of Depth and Ego-Motion From Monocular Video Using 3D Geometric Constraints (2018)
- Building Detection From Satellite Imagery Using Ensemble of Size-Specific Detectors (2018)
- On-Orbit Inspection of an Unknown, Tumbling Target Using NASA's Astrobee Robotic Free-Flyers (2021)
Tópico 6 - Competitions and Challenges in Computer Vision: The Role of NTIRE and Beyond (90 documents)
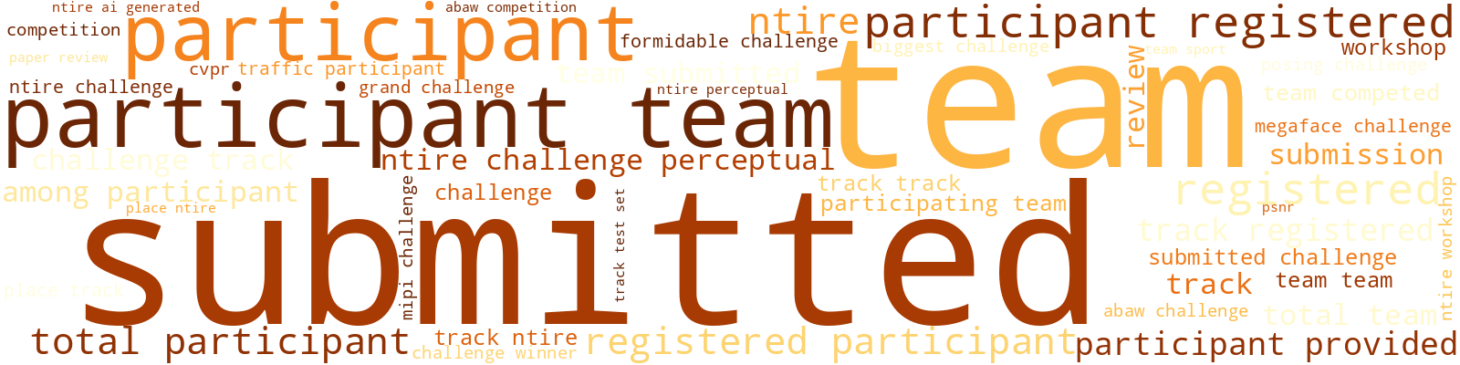
Principais autores:
- Radu Timofte (44 artigos)
- Marcos V. Conde (8 artigos)
- Radu Timofte (7 artigos)
- NTIRE 2022 Challenge on High Dynamic Range Imaging: Methods and Results (2022)
- Beyond Deep Residual Learning for Image Restoration: Persistent Homology-Guided Manifold Simplification (2017)
- NTIRE 2024 Image Shadow Removal Challenge Report (2024)
Tópico 7 - Enhancing Diffusion Models: Faster Inference and Higher Image Quality (64 documents)
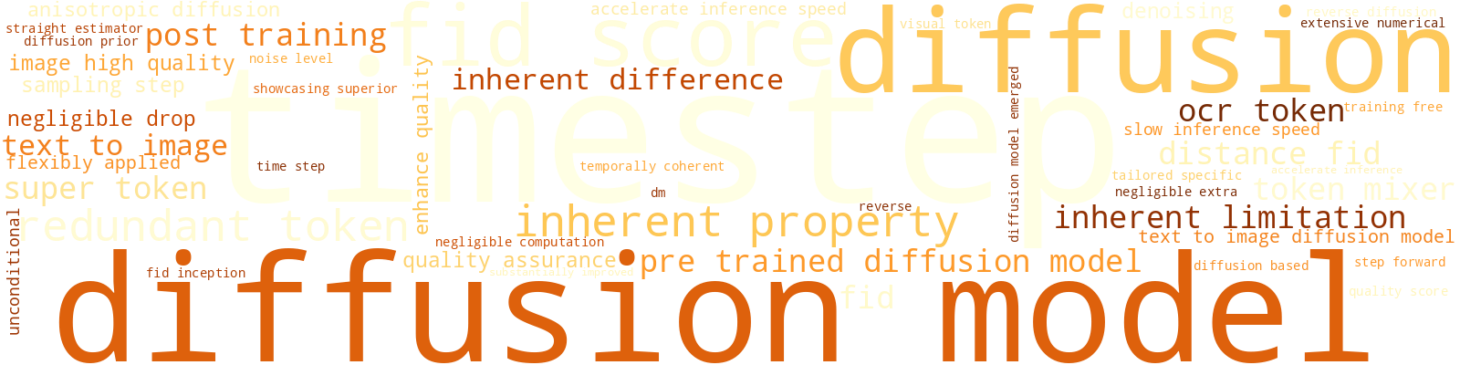
Principais autores:
- Deli Zhao (3 artigos)
- Yujun Shen (3 artigos)
- Chengyue Gong (2 artigos)
- FlowGrad: Controlling the Output of Generative ODEs With Gradients (2023)
- Plug-and-Pipeline: Efficient Regularization for Single-Step Adversarial Training (2020)
- FIANCEE: Faster Inference of Adversarial Networks via Conditional Early Exits (2023)
Tópico 8 - Intelligent Traffic Monitoring and Driver Behavior Analysis for Road Safety (58 documents)
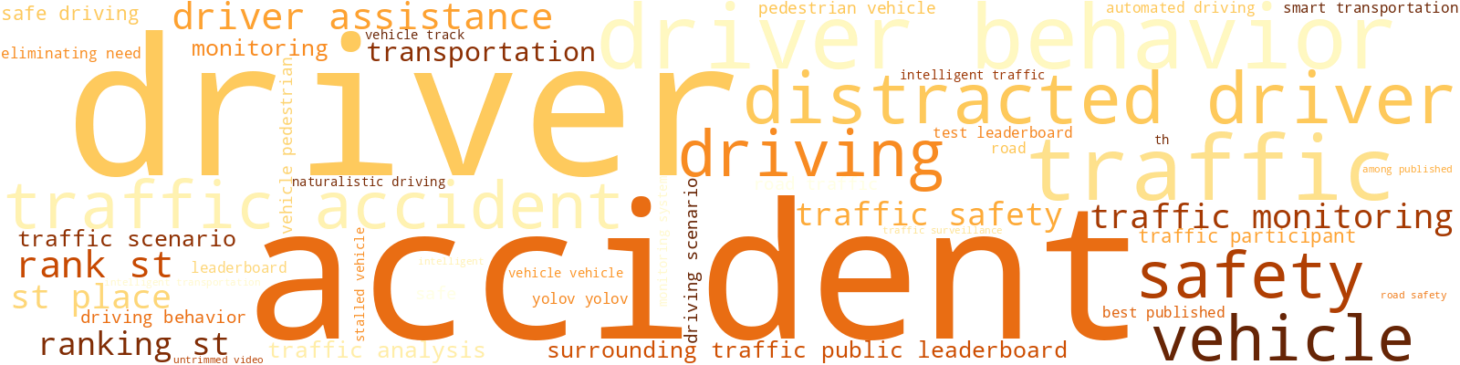
Principais autores:
- Armstrong Aboah (3 artigos)
- Fei Su (3 artigos)
- Zhe Cui (3 artigos)
- An Effective Method for Detecting Violation of Helmet Rule for Motorcyclists (2024)
- Stargazer: A Transformer-Based Driver Action Detection System for Intelligent Transportation (2022)
- Real-Time Driver Drowsiness Detection for Embedded System Using Model Compression of Deep Neural Networks (2017)
Tópico 9 - Soccer and Sports Video Analytics: Player Tracking and Game Understanding (103 documents)
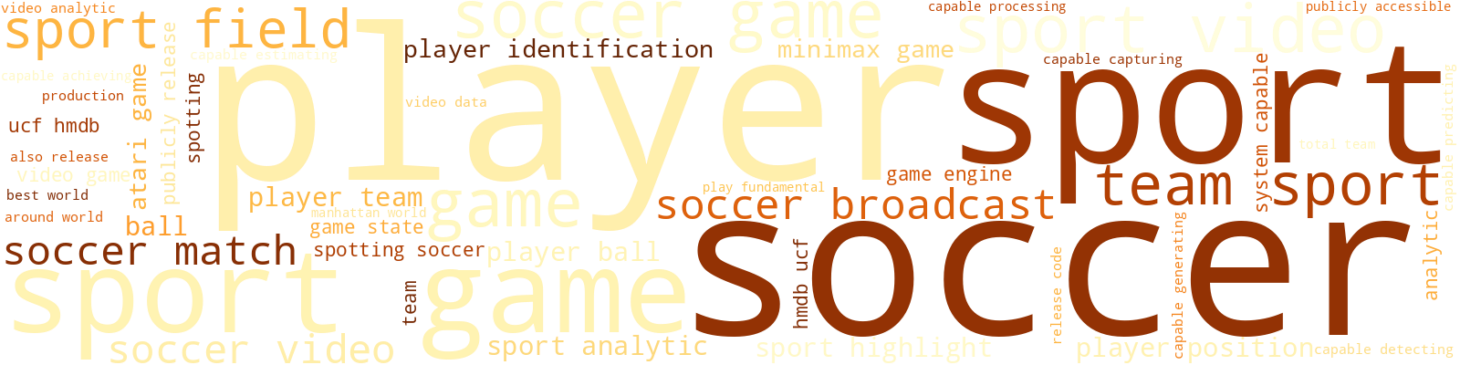
Principais autores:
- Anthony Cioppa (11 artigos)
- Marc Van Droogenbroeck (10 artigos)
- Bernard Ghanem (8 artigos)
- Spatio-Temporal Action Detection and Localization Using a Hierarchical LSTM (2020)
- SoccerNet: A Scalable Dataset for Action Spotting in Soccer Videos (2018)
- Deep 360 Pilot: Learning a Deep Agent for Piloting Through 360deg Sports Videos (2017)
Tópico 10 - Event-Based Vision: High-Speed, Low-Latency Sensing with Neuromorphic Cameras (129 documents)
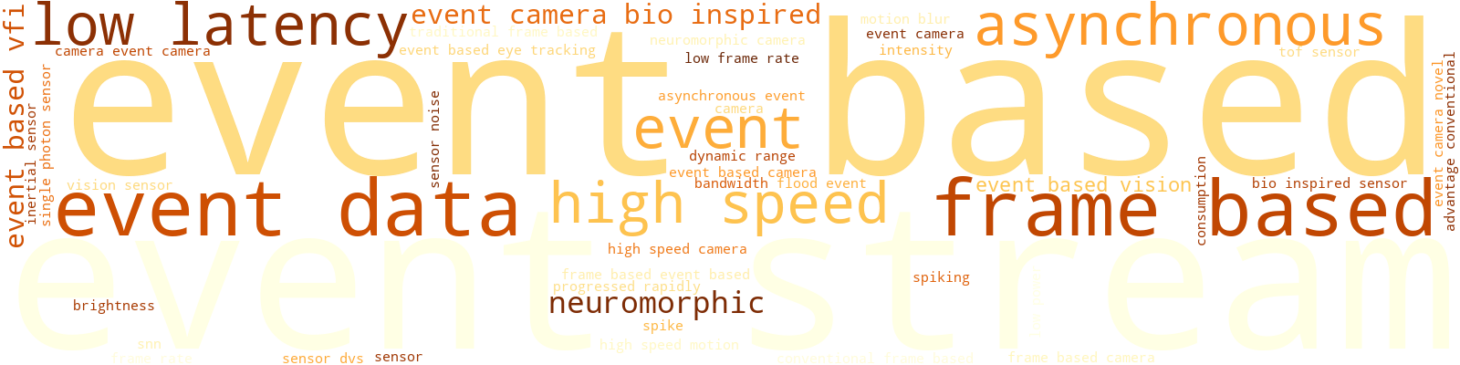
Principais autores:
- Davide Scaramuzza (11 artigos)
- Mathias Gehrig (6 artigos)
- Boxin Shi (5 artigos)
- A Voxel Graph CNN for Object Classification With Event Cameras (2022)
- Generalized Event Cameras (2024)
- EventPS: Real-Time Photometric Stereo Using an Event Camera (2024)
🧊 10 topics
{"data": [{"customdata": {"dtype": "i1", "bdata": "AQEBAQEBAQE=", "shape": "8, 1"}, "hovertemplate": "tópico=%{customdata[0]}<br>ano=%{x}<br>ocorrências (%)=%{y:.3f}<extra></extra>", "legendgroup": "1", "line": {"dash": "solid"}, "marker": {"symbol": "circle"}, "mode": "lines+markers", "name": "1", "orientation": "v", "showlegend": true, "x": {"dtype": "i2", "bdata": "4QfiB+MH5AflB+YH5wfoBw=="}, "xaxis": "x", "y": {"dtype": "f8", "bdata": "9oDZA2YPuD8TYk4TYk7DPy5/O8fHSss/aRG5pbuR1j/N5tSIhffrP1keEZpqv+o/jwTxnqx//D84iB7fhYPgPw=="}, "yaxis": "y", "type": "scatter"}, {"customdata": {"dtype": "i1", "bdata": "AgICAgICAgI=", "shape": "8, 1"}, "hovertemplate": "tópico=%{customdata[0]}<br>ano=%{x}<br>ocorrências (%)=%{y:.3f}<extra></extra>", "legendgroup": "2", "line": {"dash": "solid"}, "marker": {"symbol": "circle"}, "mode": "lines+markers", "name": "2", "orientation": "v", "showlegend": true, "x": {"dtype": "i2", "bdata": "4QfiB+MH5AflB+YH5wfoBw=="}, "xaxis": "x", "y": {"dtype": "f8", "bdata": "sVTEUhFLAUDyexnyexkCQC5/O8fHSvs/T9krrAn19D+1v65mtH76P8HIDoWtGQdAviIgBBciEUBVzK3OSMUIQA=="}, "yaxis": "y", "type": "scatter"}, {"customdata": {"dtype": "i1", "bdata": "AwMDAwMDAwM=", "shape": "8, 1"}, "hovertemplate": "tópico=%{customdata[0]}<br>ano=%{x}<br>ocorrências (%)=%{y:.3f}<extra></extra>", "legendgroup": "3", "line": {"dash": "solid"}, "marker": {"symbol": "circle"}, "mode": "lines+markers", "name": "3", "orientation": "v", "showlegend": true, "x": {"dtype": "i2", "bdata": "4QfiB+MH5AflB+YH5wfoBw=="}, "xaxis": "x", "y": {"dtype": "f8", "bdata": "9oDZA2YPuD+Y+iGY+iHYPy5/O8fHSts/0PHti4ME7T+cmIhE4wX5P76sEqjoLf0/IAQXItnI+T9VzK3OSMXoPw=="}, "yaxis": "y", "type": "scatter"}, {"customdata": {"dtype": "i1", "bdata": "BAQEBAQEBAQ=", "shape": "8, 1"}, "hovertemplate": "tópico=%{customdata[0]}<br>ano=%{x}<br>ocorrências (%)=%{y:.3f}<extra></extra>", "legendgroup": "4", "line": {"dash": "solid"}, "marker": {"symbol": "circle"}, "mode": "lines+markers", "name": "4", "orientation": "v", "showlegend": true, "x": {"dtype": "i2", "bdata": "4QfiB+MH5AflB+YH5wfoBw=="}, "xaxis": "x", "y": {"dtype": "f8", "bdata": "9oDZA2YP6D8+eSo+eSr+P23VFXiUMANAAjGEv/MeAEBiIhKNF2QJQCM7FLZmnP8/44SUPMuI/j89vgsH0ePxPw=="}, "yaxis": "y", "type": "scatter"}, {"customdata": {"dtype": "i1", "bdata": "BQUFBQUFBQU=", "shape": "8, 1"}, "hovertemplate": "tópico=%{customdata[0]}<br>ano=%{x}<br>ocorrências (%)=%{y:.3f}<extra></extra>", "legendgroup": "5", "line": {"dash": "solid"}, "marker": {"symbol": "circle"}, "mode": "lines+markers", "name": "5", "orientation": "v", "showlegend": true, "x": {"dtype": "i2", "bdata": "4QfiB+MH5AflB+YH5wfoBw=="}, "xaxis": "x", "y": {"dtype": "f8", "bdata": "9oDZA2YPyD+Y+iGY+iHYP2OfbNUVeOQ/AjGEv/Me8D8LrqN3/DDwPyhljgEOfvY/JoMsSX2tA0C1nyyBTfv7Pw=="}, "yaxis": "y", "type": "scatter"}, {"customdata": {"dtype": "i1", "bdata": "BgYGBgYGBgY=", "shape": "8, 1"}, "hovertemplate": "tópico=%{customdata[0]}<br>ano=%{x}<br>ocorrências (%)=%{y:.3f}<extra></extra>", "legendgroup": "6", "line": {"dash": "solid"}, "marker": {"symbol": "circle"}, "mode": "lines+markers", "name": "6", "orientation": "v", "showlegend": true, "x": {"dtype": "i2", "bdata": "4QfiB+MH5AflB+YH5wfoBw=="}, "xaxis": "x", "y": {"dtype": "f8", "bdata": "77S60+pOB0DhCH/hCH8BQELrjQzFu/g/HGkRuaW78T8j1cmZzanxP/SPD4zsUPg/BITgQiQb+T+61RmpmFvtPw=="}, "yaxis": "y", "type": "scatter"}, {"customdata": {"dtype": "i1", "bdata": "BwcHBwcHBwc=", "shape": "8, 1"}, "hovertemplate": "tópico=%{customdata[0]}<br>ano=%{x}<br>ocorrências (%)=%{y:.3f}<extra></extra>", "legendgroup": "7", "line": {"dash": "solid"}, "marker": {"symbol": "circle"}, "mode": "lines+markers", "name": "7", "orientation": "v", "showlegend": true, "x": {"dtype": "i2", "bdata": "4QfiB+MH5AflB+YH5wfoBw=="}, "xaxis": "x", "y": {"dtype": "f8", "bdata": "qYilIpaK8D8TYk4TYk7zP2OfbNUVeOQ/0PHti4ME7T/N5tSIhffrP1w6DXcvq+Q/PIRNAY52+j/hIHp8Fw7wPw=="}, "yaxis": "y", "type": "scatter"}, {"customdata": {"dtype": "i1", "bdata": "CAgICAgICAg=", "shape": "8, 1"}, "hovertemplate": "tópico=%{customdata[0]}<br>ano=%{x}<br>ocorrências (%)=%{y:.3f}<extra></extra>", "legendgroup": "8", "line": {"dash": "solid"}, "marker": {"symbol": "circle"}, "mode": "lines+markers", "name": "8", "orientation": "v", "showlegend": true, "x": {"dtype": "i2", "bdata": "4QfiB+MH5AflB+YH5wfoBw=="}, "xaxis": "x", "y": {"dtype": "f8", "bdata": "uSDjgowL4j/RleTQleTgP3Dn+Fhpw+I/aRG5pbuR5j+1v65mtH7qP/eySqCitwBAmwIc7fRIAED5LhxEj+/2Pw=="}, "yaxis": "y", "type": "scatter"}, {"customdata": {"dtype": "i1", "bdata": "CQkJCQkJCQk=", "shape": "8, 1"}, "hovertemplate": "tópico=%{customdata[0]}<br>ano=%{x}<br>ocorrências (%)=%{y:.3f}<extra></extra>", "legendgroup": "9", "line": {"dash": "solid"}, "marker": {"symbol": "circle"}, "mode": "lines+markers", "name": "9", "orientation": "v", "showlegend": true, "x": {"dtype": "i2", "bdata": "4QfiB+MH5AflB+YH5wfoBw=="}, "xaxis": "x", "y": {"dtype": "f8", "bdata": "9oDZA2YPyD8TYk4TYk6zPy5/O8fHSrs/NqGesldY0z+1v65mtH7aP76sEqjoLd0/mwIc7fRI8D9eOIge34XbPw=="}, "yaxis": "y", "type": "scatter"}, {"customdata": {"dtype": "i1", "bdata": "CgoKCgoKCgo=", "shape": "8, 1"}, "hovertemplate": "tópico=%{customdata[0]}<br>ano=%{x}<br>ocorrências (%)=%{y:.3f}<extra></extra>", "legendgroup": "10", "line": {"dash": "solid"}, "marker": {"symbol": "circle"}, "mode": "lines+markers", "name": "10", "orientation": "v", "showlegend": true, "x": {"dtype": "i2", "bdata": "4QfiB+MH5AflB+YH5wfoBw=="}, "xaxis": "x", "y": {"dtype": "f8", "bdata": "2FBeQ3kNFUAdk/Uck/UMQGnDMpe/nQNAdq1/ohRgB0CKSDRekKX/P7+6kBbLI/o/lYMGxlBk9j+waT9ZApvqPw=="}, "yaxis": "y", "type": "scatter"}], "layout": {"xaxis": {"anchor": "y", "domain": [0.0, 1.0], "title": {"text": "ano"}}, "yaxis": {"anchor": "x", "domain": [0.0, 1.0], "title": {"text": "ocorrências (%)"}}, "legend": {"title": {"text": "tópico"}, "tracegroupgap": 0}}}
Os tópicos a seguir estão listados na ordem em que tiveram a maior diminuição de artigos no último ano.
Tópico 1 - Self-Supervised Pretraining: Masked Models and Their Impact on Downstream Vision Tasks (115 documents)
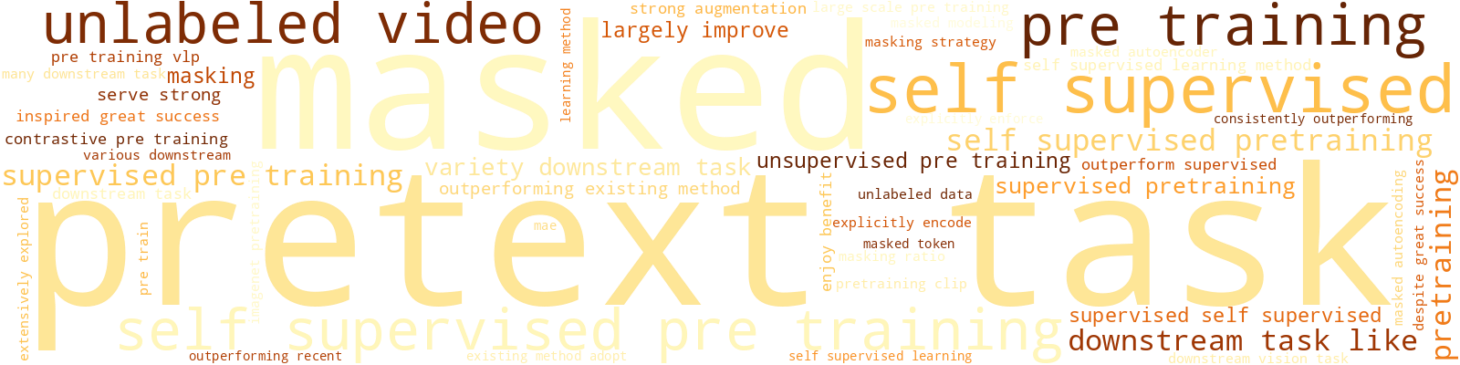
Principais autores:
- Yu Qiao (9 artigos)
- Ishan Misra (4 artigos)
- Ross Girshick (4 artigos)
- Solving Masked Jigsaw Puzzles with Diffusion Vision Transformers (2024)
- Learning Features by Watching Objects Move (2017)
- Self-Supervised Learning with Generative Adversarial Networks for Electron Microscopy (2024)
Tópico 2 - Vision-Language Models: Aligning Text and Image for Cross-Modal Understanding (432 documents)
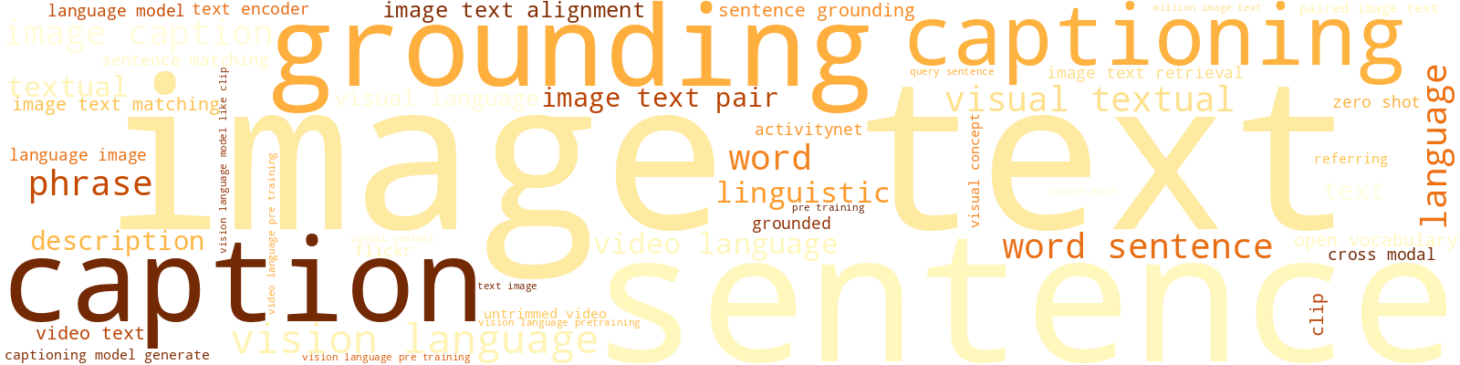
Principais autores:
- Lijuan Wang (9 artigos)
- Mike Zheng Shou (7 artigos)
- Ying Shan (7 artigos)
- Image Search With Text Feedback by Visiolinguistic Attention Learning (2020)
- ULIP: Learning a Unified Representation of Language, Images, and Point Clouds for 3D Understanding (2023)
- MobileCLIP: Fast Image-Text Models through Multi-Modal Reinforced Training (2024)
Tópico 3 - Semi-Supervised Learning: Leveraging Unlabeled Data for Improved Model Performance (179 documents)
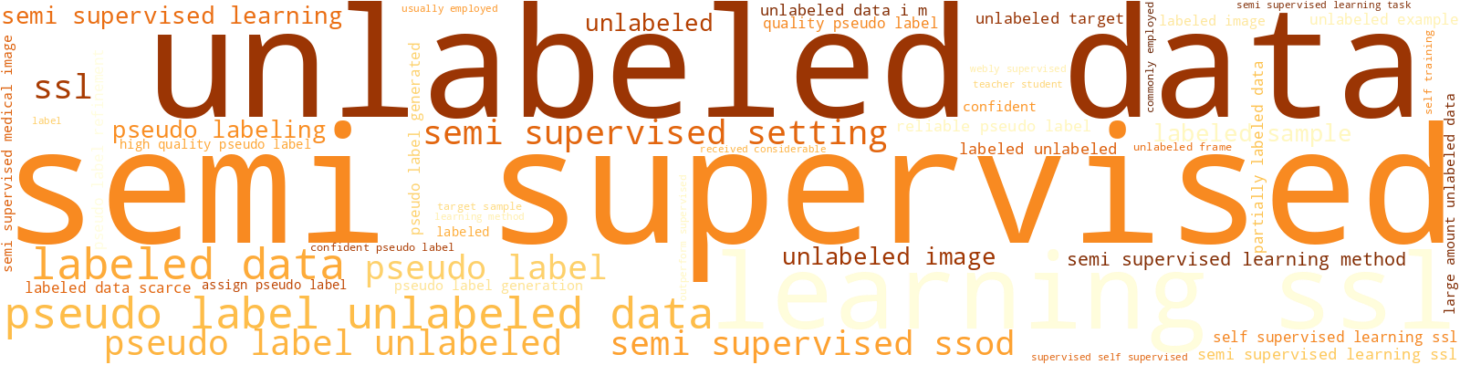
Principais autores:
- Jingdong Wang (4 artigos)
- Lei Qi (4 artigos)
- Yinghuan Shi (4 artigos)
- Decoupled Pseudo-labeling for Semi-Supervised Monocular 3D Object Detection (2024)
- Semi-Supervised Learning With Scarce Annotations (2020)
- Phase Consistent Ecological Domain Adaptation (2020)
Tópico 4 - Domain Adaptation: Bridging the Gap Between Source and Target Domains (323 documents)
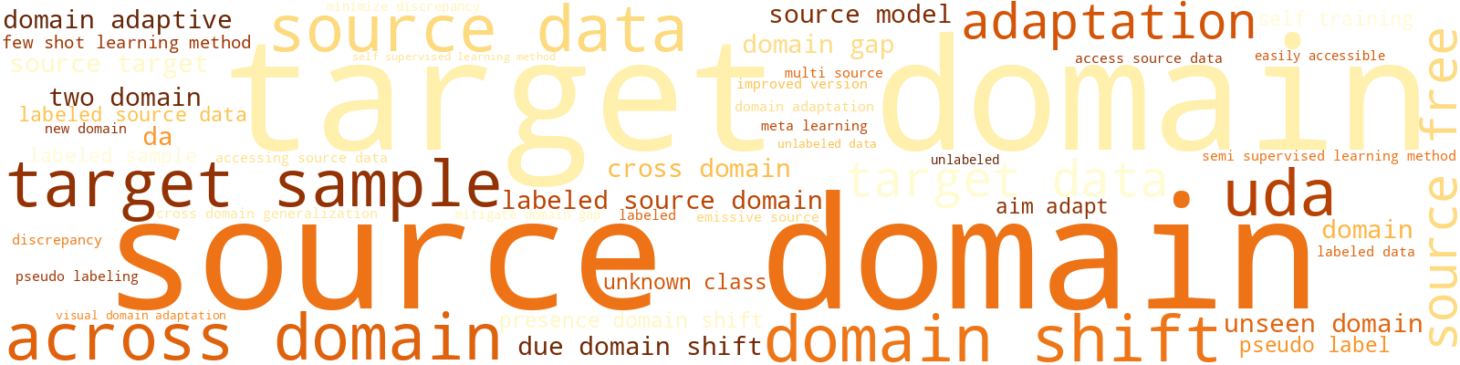
Principais autores:
- Luc Van Gool (8 artigos)
- Dengxin Dai (7 artigos)
- Wen Li (7 artigos)
- Contrastive Domain Adaptation (2021)
- DAMSL: Domain Agnostic Meta Score-Based Learning (2021)
- Spatio-temporal Contrastive Domain Adaptation for Action Recognition (2021)
Tópico 5 - 3D Object Detection: Advancements in Lidar and Monocular Approaches for Autonomous Vehicles (217 documents)
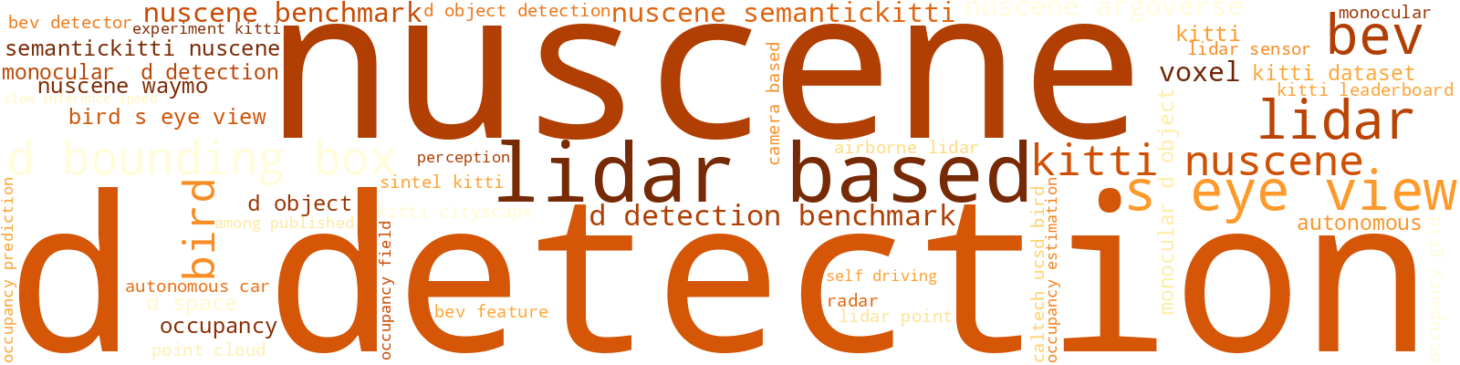
Principais autores:
- Jie Zhou (7 artigos)
- Jiwen Lu (6 artigos)
- Yuexin Ma (6 artigos)
- GAFusion: Adaptive Fusing LiDAR and Camera with Multiple Guidance for 3D Object Detection (2024)
- SparseOcc: Rethinking Sparse Latent Representation for Vision-Based Semantic Occupancy Prediction (2024)
- IS-Fusion: Instance-Scene Collaborative Fusion for Multimodal 3D Object Detection (2024)
Tópico 6 - Weakly Supervised Object Segmentation: Balancing Annotations and Performance (244 documents)
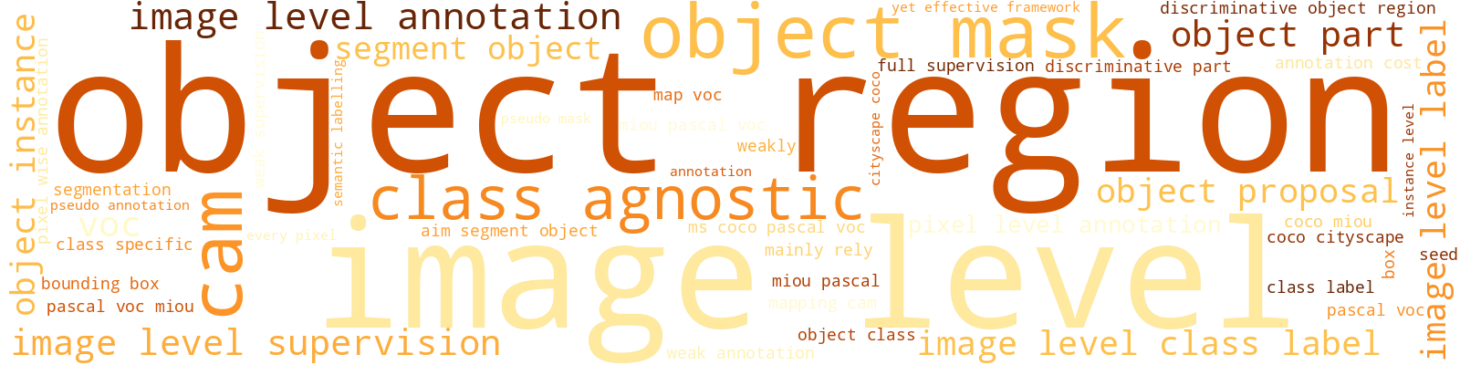
Principais autores:
- Junwei Han (5 artigos)
- Yunchao Wei (5 artigos)
- Bingfeng Zhang (4 artigos)
- CascadePSP: Toward Class-Agnostic and Very High-Resolution Segmentation via Global and Local Refinement (2020)
- SimpSON: Simplifying Photo Cleanup With Single-Click Distracting Object Segmentation Network (2023)
- Distilling Self-Supervised Vision Transformers for Weakly-Supervised Few-Shot Classification & Segmentation (2023)
Tópico 7 - Image Relighting: Enhancing Lighting and Material Effects in Digital Rendering (167 documents)
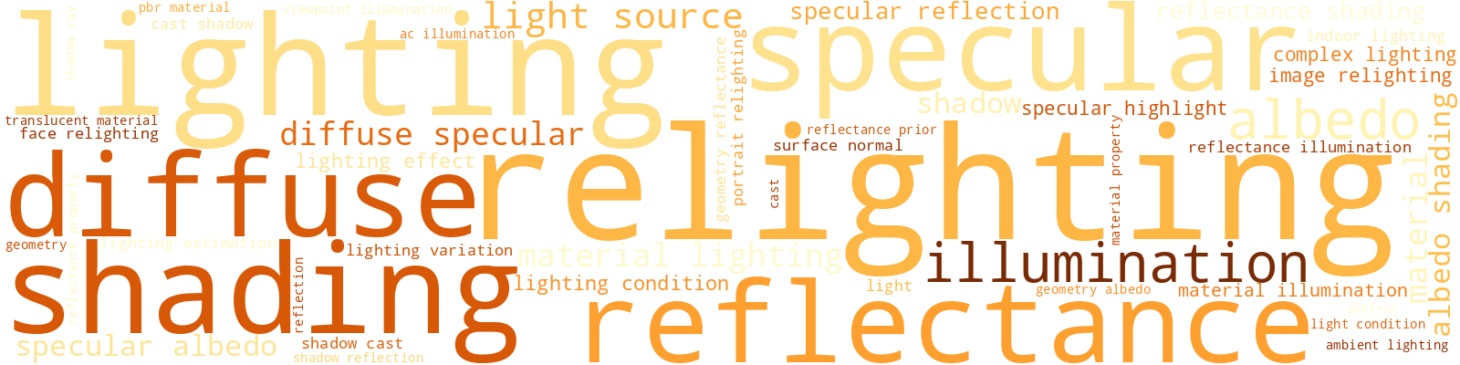
Principais autores:
- Boxin Shi (11 artigos)
- Kalyan Sunkavalli (7 artigos)
- Noah Snavely (6 artigos)
- Uncalibrated Photometric Stereo Under Natural Illumination (2018)
- Dynamic Fluid Surface Reconstruction Using Deep Neural Network (2020)
- Monocular Reconstruction of Neural Face Reflectance Fields (2021)
Tópico 8 - Large Kernel Convolutions and Self-Attention Mechanisms in Vision Transformers (209 documents)
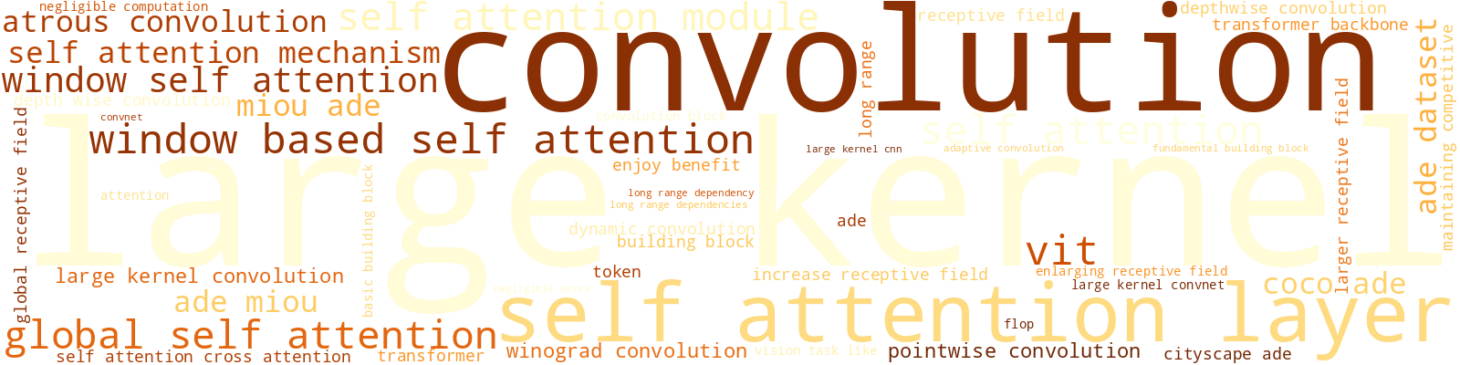
Principais autores:
- Xiangyu Zhang (6 artigos)
- Chang Xu (5 artigos)
- Yu Qiao (5 artigos)
- PatchFormer: An Efficient Point Transformer With Patch Attention (2022)
- UniRepLKNet: A Universal Perception Large-Kernel ConvNet for Audio Video Point Cloud Time-Series and Image Recognition (2024)
- Gaussian Context Transformer (2021)
Tópico 9 - 3D-Aware Image Synthesis with GANs for High-Fidelity and Controllable Rendering (71 documents)
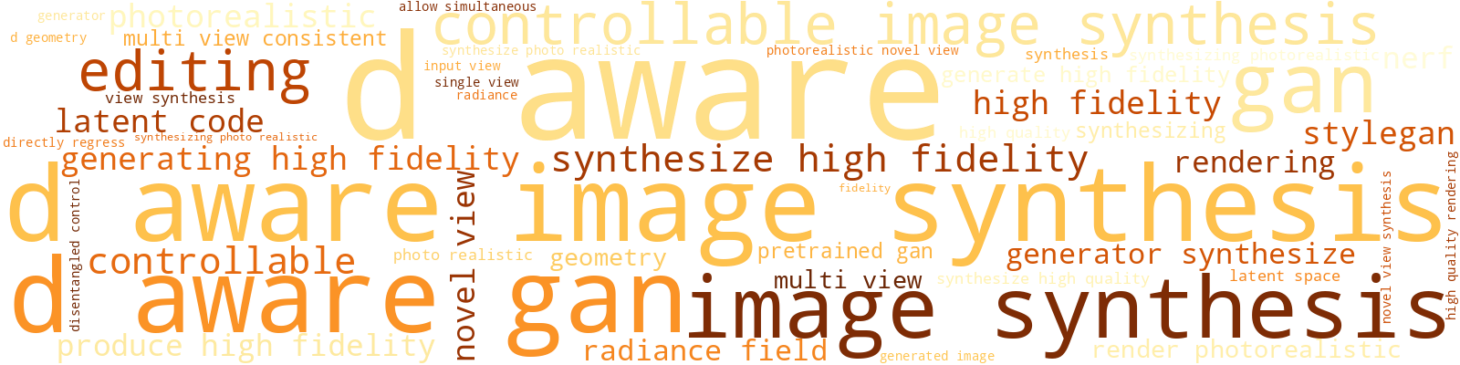
Principais autores:
- Gordon Wetzstein (4 artigos)
- Jiajun Wu (4 artigos)
- Sida Peng (4 artigos)
- DisCoScene: Spatially Disentangled Generative Radiance Fields for Controllable 3D-Aware Scene Synthesis (2023)
- NeuralField-LDM: Scene Generation With Hierarchical Latent Diffusion Models (2023)
- Lift3D: Synthesize 3D Training Data by Lifting 2D GAN to 3D Generative Radiance Field (2023)
Tópico 10 - Efficient Solving of Non-Convex Problems with Outlier Rejection and Relaxation Techniques (356 documents)
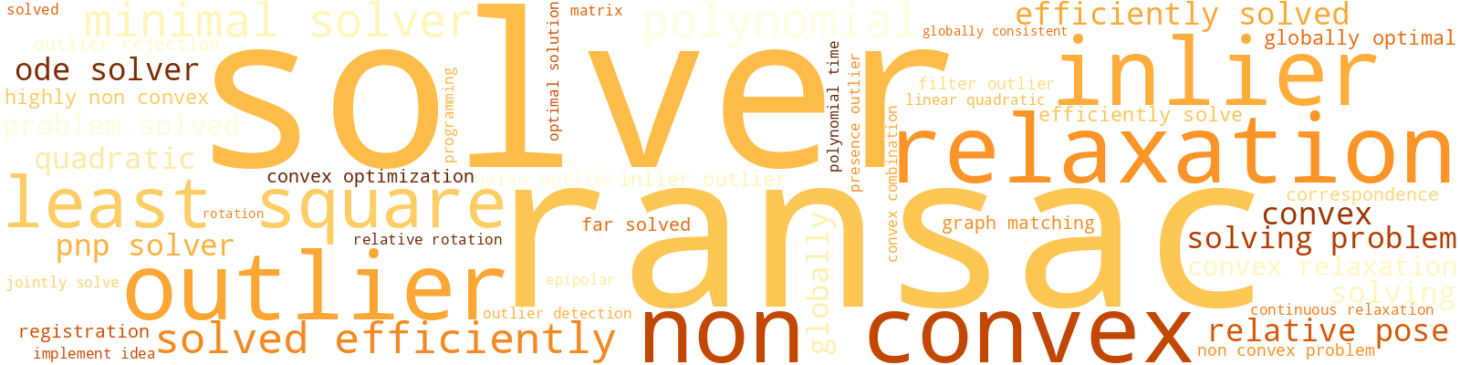
Principais autores:
- Daniel Barath (13 artigos)
- Daniel Cremers (13 artigos)
- Viktor Larsson (10 artigos)
- Sliced Optimal Partial Transport (2023)
- 3D Registration With Maximal Cliques (2023)
- A Rotation-Translation-Decoupled Solution for Robust and Efficient Visual-Inertial Initialization (2023)
Conclusão
Nesta análise, exploramos as tendências e mudanças nos tópicos de pesquisa da comunidade CVPR ao longo dos últimos anos. Os dados revelam um cenário dinâmico, com algumas áreas apresentando crescimento significativo, enquanto outras mostram uma queda de interesse. Isso reflete a natureza evolutiva da pesquisa em inteligência artificial e a busca contínua por inovação e aprimoramento em diversos domínios.
Os tópicos mais quentes – que vão desde LLMs multimodais com fine-tuning por instrução para compreensão visão-linguagem até os avanços rápidos na edição orientada por texto, síntese com consciência do 3D e visão baseada em eventos – evidenciam um forte impulso na integração das modalidades, na criação de modelos generativos mais controláveis e na abordagem das crescentes demandas de aplicações reais. Pesquisadores estão cada vez mais empenhados em melhorar a integração entre linguagem e visão para possibilitar um raciocínio mais eficaz, lidar melhor com ambiguidades (como alucinações) e aprimorar o desempenho tanto em contextos criativos quanto em ambientes críticos para a segurança.
Em contraste, os tópicos mais frios – tais como o pré-treinamento auto-supervisionado, o alinhamento tradicional visão-linguagem, o aprendizado semi-supervisionado, a adaptação de domínio e até mesmo a detecção clássica de objetos 3D – indicam áreas onde técnicas bem estabelecidas parecem ter atingido um platô. Enquanto esses métodos lançaram as bases para os avanços atuais, sua evolução desacelerou em favor de abordagens mais recentes. Técnicas que outrora foram inovadoras estão sendo revisitadas com o intuito de integrá-las a sistemas mais abrangentes, mas seu apelo isolado diminuiu à medida que a comunidade se volta para soluções end-to-end, multimodais e específicas para cada tarefa.
Em conjunto, essas tendências sugerem que o campo está se direcionando para modelos mais holísticos e integrados, que não apenas expandem os limites do que os sistemas automatizados podem gerar ou analisar, mas também proporcionam maior confiabilidade e controle em aplicações do mundo real. À medida que a indústria continua a explorar a fusão de texto, imagem e até dados de sensores, a próxima onda de inovação provavelmente será impulsionada por sistemas que aprendem simultaneamente a partir de múltiplas modalidades, enquanto aproveitam métodos robustos já estabelecidos como alicerce.
Essa evolução ressalta a natureza vibrante da pesquisa em inteligência artificial, onde métodos consagrados oferecem uma base sólida, ao mesmo tempo em que as técnicas emergentes prometem remodelar o futuro da inteligência artificial.
Gostou de Ler este Artigo?
Aqui estão alguns artigos relacionados que você pode gostar de ler: